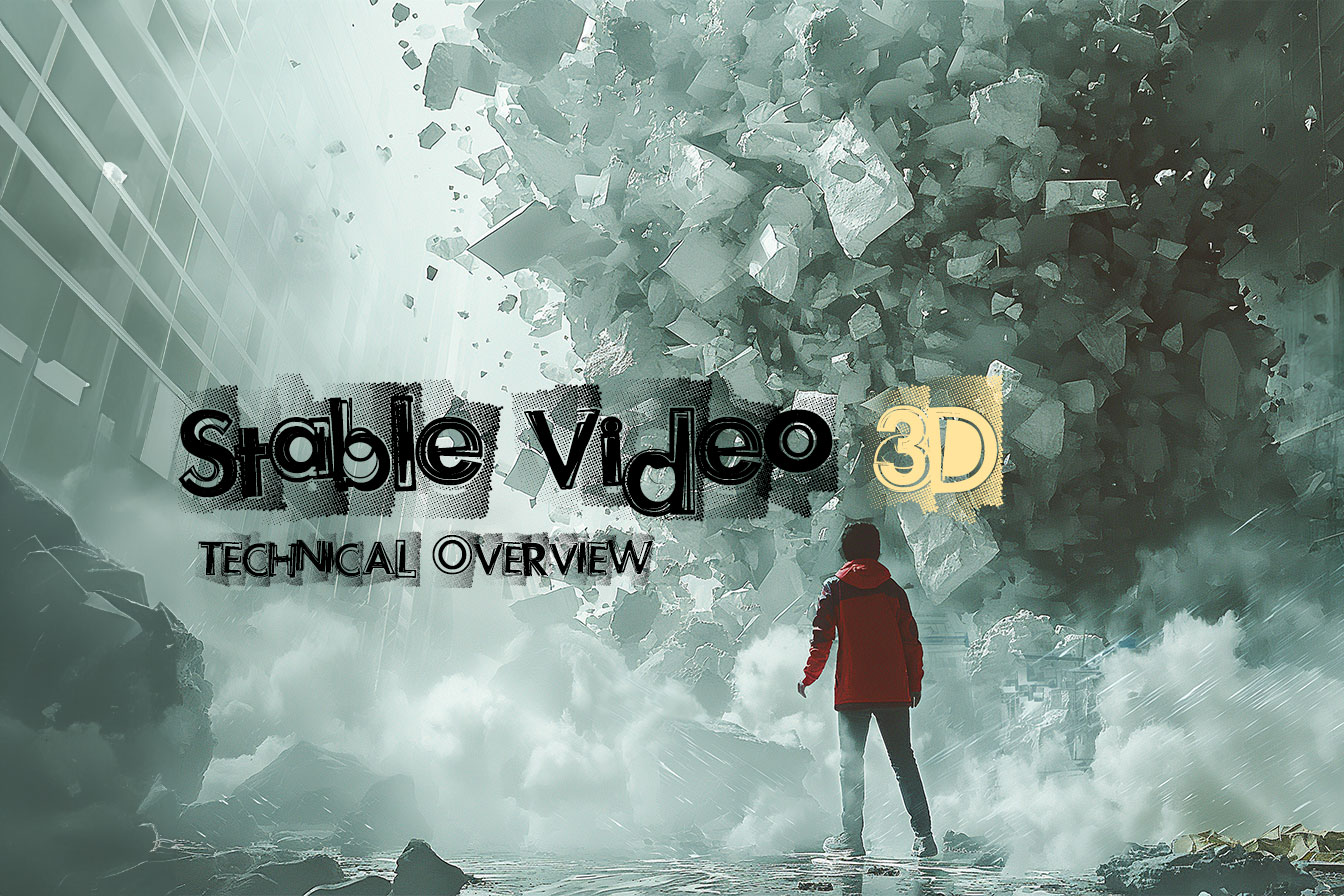
Stable Video 3D Early Preview: Technical Overview
The development of Stable Video 3D (SV3D) marks a significant advancement in 3D content generation. By enabling the creation of high-quality 3D models from single images, SV3D offers a practical solution for the challenges faced in the production of digital media. This technical overview provides an insight into the mechanics of SV3D and its role in improving the efficiency of the creative production pipeline.
SV3D’s generative model is a key innovation, extending the capabilities of Stable Video Diffusion to transform the process of 3D modeling. With SV3D, creators can achieve consistent and realistic 3D representations, which are essential for a variety of applications across multiple industries.
Table of Contents
The Technology Behind SV3D
Stable Video 3D (SV3D) represents a significant leap in 3D content generation, offering a unique approach to creating detailed and consistent 3D models from single images. This section outlines the technical foundation of SV3D and its two main variants, SV3D_u and SV3D_p.
Generative Models
SV3D is built on generative models, which are a class of artificial intelligence that can create new data instances that resemble the training data. These models are particularly adept at understanding and replicating complex patterns, making them ideal for tasks like novel view synthesis.
SV3D Variants
- SV3D_u: This variant is designed to generate orbital videos from single image inputs without the need for camera conditioning. It’s particularly useful for applications where the camera path does not need to be predefined.
- SV3D_p: Extending the capabilities of SV3D_u, SV3D_p allows for the generation of 3D video along specified camera paths. This is crucial for scenarios where precise camera movement is required to capture the desired views.
Multi-View Consistency
A core aspect of SV3D’s technology is its focus on multi-view consistency. This ensures that the generated 3D models remain stable and realistic across different viewpoints. To achieve this, SV3D optimizes 3D Neural Radiance Fields (NeRF) and mesh representations, which are pivotal in maintaining the integrity of the 3D models.
Enhancing Mesh Quality
The quality of 3D meshes generated directly from novel views is enhanced through SV3D’s advanced algorithms. This results in more accurate and lifelike 3D representations, which are essential for creating immersive experiences in digital media.
Learn More about SV3D | Learn More about on HuggingFace
Technical Specifications and Performance of SV3D
The deployment and operation of Stable Video 3D (SV3D) demand a specific set of technical specifications to ensure optimal performance. This section outlines the necessary system requirements, performance metrics, and the overall efficiency of SV3D.
System Requirements
To utilize SV3D effectively, certain hardware and software requirements must be met:
- Hardware: A robust GPU with ample VRAM is recommended to handle the intensive computational tasks associated with 3D model generation.
- Software: SV3D requires compatible software environments that support its generative models, typically involving machine learning libraries and frameworks.
Minimum System Requirements
- Operating System: Windows 10 or 11.
- Processor: Intel Core i5 (8th generation or greater).
- RAM: 8GB or more.
- Graphics Card: Nvidia GeForce GTX 9 or 10 series (or comparable Radeon).
- VRAM: At least 4GB.
- Storage: 12GB or more install space, SSD recommended.
Optimal System Requirements
- Operating System: Windows 10 or 11.
- Processor: Intel Core i7 or i9 (9th generation or greater).
- RAM: 16GB or more.
- Graphics Card: Nvidia GeForce RTX 3060 or greater.
- VRAM: 10GB or more.
- Storage: 1TB or more, with a combination of SSD for software and HDD for storage.
Performance Metrics
SV3D’s performance can be evaluated based on several key metrics:
- Processing Speed: The time taken to generate 3D models from input images is a critical factor, especially when dealing with large datasets or real-time applications.
- Quality of Output: The fidelity of the generated 3D models is assessed in terms of detail, texture, and consistency across different views.
Efficiency
The efficiency of SV3D is a testament to its advanced algorithms and optimization techniques. By minimizing computational overhead and maximizing output quality, SV3D stands out as a highly efficient tool for 3D content generation.
In the following section, we will explore the models that power SV3D, including their architecture and the training process that enables their remarkable capabilities.
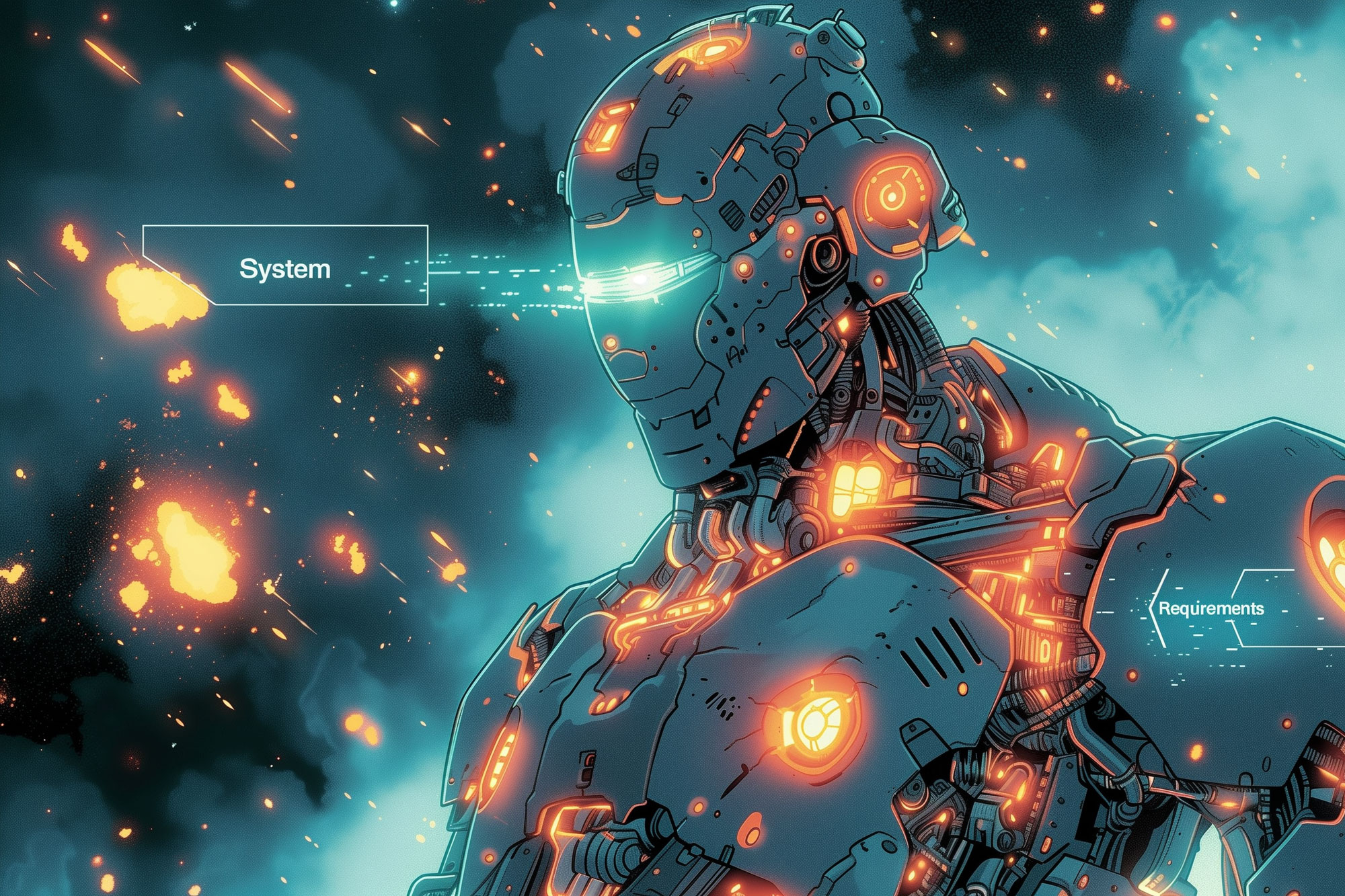
Stable Diffusion, one of the most popular AI art-generation tools, offers impressive results but demands a robust system. Whether you’re a creative artist or an enthusiast, understanding the System Requirements for Stable Diffusion is important for efficient and smooth operation. In this comprehensive guide, we’ll go deep into the specifics of running Stable Diffusion effectively,…
The Models Powering Stable Video 3D
The efficacy of Stable Video 3D (SV3D) is largely attributed to its robust models, which are the result of a meticulous training process. This section provides an overview of the architecture of these models and the training they undergo to perform their tasks.
Model Architecture
SV3D models are built on a deep neural network architecture that is specifically designed for processing and generating 3D content. The architecture includes:
- Convolutional Neural Networks (CNNs): Utilized for their ability to handle image data efficiently.
- Recurrent Neural Networks (RNNs): Employed to maintain temporal consistency in video generation.
- Generative Adversarial Networks (GANs): Used to refine the quality of the generated 3D models.
Training Process
The training of SV3D models involves several stages:
- Data Collection: A diverse dataset of images and videos is compiled to cover a wide range of scenarios and objects.
- Preprocessing: The data is cleaned and formatted to ensure uniformity, which is crucial for the learning process.
- Supervised Learning: The models are trained using labeled data, allowing them to learn the correlation between input images and the desired 3D output.
- Unsupervised Learning: To enhance their generative capabilities, the models also undergo training with unlabeled data, learning to infer 3D structures from 2D images.
- Fine-Tuning: The models are further refined through fine-tuning, where they are exposed to new data or specific tasks to improve their performance.
Performance Evaluation
After training, the models are evaluated based on their ability to generate accurate and stable 3D models. This involves:
- Validation: Using a separate set of data to test the models’ performance and ensure they generalize well to new data.
- Benchmarking: Comparing the models’ output against established standards and metrics in the field of 3D content generation.
The next section will discuss the practical applications of SV3D, showcasing its versatility and potential use cases in various industries.
Practical Applications of Stable Video 3D
The introduction of Stable Video 3D (SV3D) into the market opens up a plethora of practical applications that can benefit various sectors. This section highlights the versatility of SV3D and its potential to revolutionize the way we interact with digital content.
Industry Use Cases
SV3D’s ability to generate high-quality 3D models from single images has significant implications for several industries:
- Gaming: Game developers can use SV3D to create immersive 3D environments and characters, enhancing the gaming experience.
- Film and Animation: Filmmakers and animators can utilize SV3D to produce detailed 3D assets for use in visual effects and animated features.
- Virtual and Augmented Reality: SV3D can provide a quick and efficient way to create 3D models for VR and AR experiences, making them more accessible and realistic.
- Architecture and Engineering: Professionals in these fields can benefit from SV3D’s capabilities to visualize structures and prototypes in 3D space.
User Experience
The user experience with SV3D is designed to be as intuitive and user-friendly as possible:
- Interface: The SV3D interface is streamlined to facilitate easy navigation and operation, even for users with limited technical expertise.
- Workflow Integration: SV3D can be integrated into existing workflows, allowing for seamless transition from 2D to 3D content creation.
While the model itself is a backend technology, there is a user interface available for Stable Video 3D which is part of the StableSwarmUI, which is a modular web-user-interface designed to make powerful tools like SV3D easily accessible and user-friendly. The interface is currently in beta status, which means it’s functional and available for general use, but it may still have some bugs and quality-of-life improvements to be worked out. This interface allows users to interact with SV3D and other models, providing a practical way to utilize these advanced AI tools.
Learn how to install StableSwarmUI below:
Community and Support
SV3D is supported by a robust community and a range of support resources:
- Documentation: Comprehensive documentation is provided to help users understand and utilize all features of SV3D.
- Forums and Online Communities: Users can connect with others to share tips, tricks, and advice on getting the most out of SV3D.
Join the Stable Diffusion Discord.
Advantages of Video Diffusion
By adapting our Stable Video Diffusion image-to-video diffusion model with the addition of camera path conditioning, Stable Video 3D is able to generate multi-view videos of an object. The use of video diffusion models, in contrast to image diffusion models as used in Stable Zero123, provides major benefits in generalization and view-consistency of generated outputs. Additionally, we propose improved 3D optimization leveraging this powerful capability of Stable Video 3D to generate arbitrary orbits around an object. By further implementing these techniques with disentangled illumination optimization as well as a new masked score distillation sampling loss function, Stable Video 3D is able to reliably output quality 3D meshes from single image inputs.
See the technical report here for more details on the Stable Video 3D models and experimental comparisons.
Novel-View Generation
Stable Video 3D introduces significant advancements in 3D generation, particularly in novel view synthesis (NVS). Unlike previous approaches that often grapple with limited perspectives and inconsistencies in outputs, Stable Video 3D is able to deliver coherent views from any given angle with proficient generalization. This capability not only enhances pose-controllability, but also ensures consistent object appearance across multiple views, further improving critical aspects of realistic and accurate 3D generations.
Stabilty AI
Challenges and Future Directions of Stable Video 3D
As with any cutting-edge technology, Stable Video 3D (SV3D) faces its own set of challenges and limitations. However, the ongoing research and development efforts promise to address these issues and pave the way for future advancements. This section discusses the current hurdles and the potential trajectory of SV3D’s evolution.
Addressing Current Limitations
While SV3D has made impressive strides in 3D content generation, there are still challenges to be overcome:
- Data Requirements: The need for large and diverse datasets to train the models can be a limiting factor, especially for less common subjects.
- Computational Resources: The intensive computational demands of SV3D may limit its accessibility to users with high-end hardware.
- Realism and Accuracy: Ensuring the generated 3D models accurately reflect real-world physics and aesthetics remains an ongoing challenge.
Overcoming the Hurdles
Efforts are underway to mitigate these limitations:
- Data Efficiency: Research into more data-efficient training methods could reduce the need for extensive datasets.
- Optimization: Continuous improvements in the model’s algorithms aim to lower the computational load, making SV3D more accessible.
- Quality Enhancement: Advances in neural rendering and mesh generation techniques are expected to improve the realism and accuracy of the models.
Anticipated Developments
Looking ahead, SV3D is poised for several exciting developments:
- User-Friendly Tools: The refinement of user interfaces and tools will make SV3D more approachable for a broader range of users.
- Integration with Other Technologies: Combining SV3D with other AI advancements could lead to more comprehensive and powerful creative suites.
- Expansion of Use Cases: As SV3D matures, its applications are expected to extend beyond current industries, potentially impacting fields like education, healthcare, and more.
Conclusion: Envisioning the Future of 3D Animation
What will the 3D animation industry look like in the near future? Will AI-driven tools like SV3D become the new standard, automating the entire pipeline and freeing creators to focus solely on the art of storytelling? Could we witness the birth of a completely new pipeline that redefines our approach to animation, rendering traditional methods obsolete?
Or perhaps, SV3D will serve as a powerful adjunct to the current toolkit, enhancing and streamlining workflows without completely displacing the skills and techniques honed by animators over decades.
Is SV3D heralding an era where the boundaries between creator and creation blur, where AI becomes a collaborator rather than just a tool? Could this be the dawn of a new creative renaissance, one where the limitations of technical execution no longer constrain the imagination?
What do you envision for the future of 3D animation? Will AI be the brush with which we paint new worlds, or will it be the canvas that amplifies our existing art?
Tags And Categories
Share this post
One response to “Stable Video 3D Early Preview: Technical Overview”
-
Thanks for post!
Leave a Reply