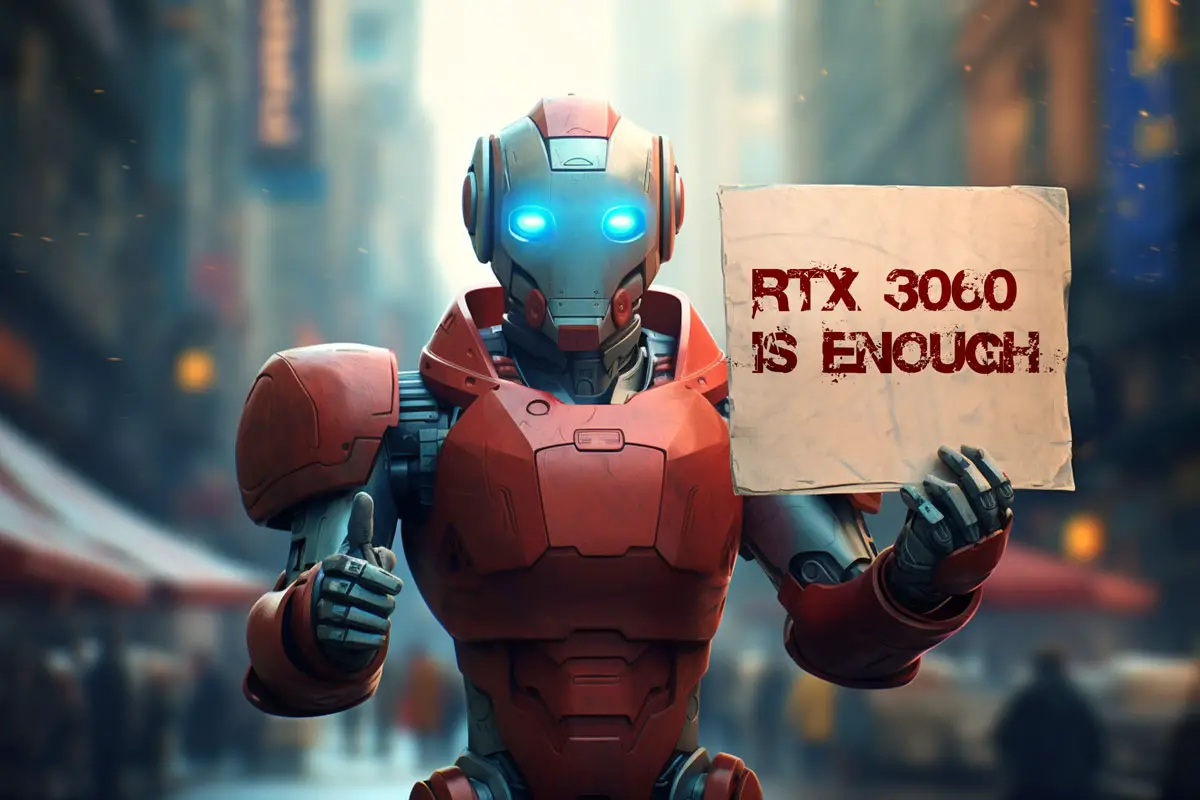
Is RTX 3060 Good for Stable Diffusion?
Is RTX 3060 Good for Stable Diffusion? If you’re considering the NVIDIA RTX 3060 for Stable Diffusion, you’re heading in the right direction. Artificial intelligence (AI) and image generation has witnessed incredible advancements in recent years. From deep learning models to generative adversarial networks (GANs), the quest for creating realistic and flawless images has been relentless. Among the essential tools is the graphics processing unit (GPU), which plays a pivotal role in accelerating computations and rendering lifelike visuals.
In this blog post, we will learn about the effectiveness and suitability of the NVIDIA GeForce RTX 3060 for Stable Diffusion. Whether you’re an new-age aspiring digital artist, a researcher, or simply curious about the intersection of hardware and creativity, read on to discover why the RTX 3060 deserves a spot in your GPU lineup.
Table of Contents
The NVIDIA RTX 3060 is a well-regarded graphics card that boasts 12GB of GDDR6 VRAM, which is more than sufficient to run Stable Diffusion tasks effectively. The card’s generous VRAM, combined with its powerful GPU architecture, allows it to manage complex image generation tasks and deliver impressive results.
In terms of Stable Diffusion performance, the RTX 3060 should offer a significant advantage over lower-end or older graphics cards. Its advanced features, such as real-time ray tracing and AI-powered DLSS, can potentially enhance the quality of generated images, depending on the specific Stable Diffusion model you’re using.
1. Performance
The RTX 3060 boasts impressive performance metrics, making it a compelling choice for Stable Diffusion tasks. Here’s what you need to know:
CUDA Cores:
With 3584 CUDA cores, the RTX 3060 flexes its computational muscles. These cores handle parallel processing, enabling faster model training and inference.
Tensor Cores:
The inclusion of Tensor Cores enhances AI workloads. These specialized cores accelerate matrix operations, crucial for neural network computations.
Clock Speeds:
The base clock speed of 1320 MHz and a boost clock of 1777 MHz ensure snappy performance across various tasks.
2. VRAM Capacity
When it comes to Stable Diffusion, the VRAM (Video Random Access Memory) of a GPU plays a crucial role. Let’s go deeper into why the 12 GB of GDDR6 memory in the RTX 3060 matters for pushing the limits.
Handling Large Image Tensors
Stable Diffusion algorithms involve complex computations on image tensors. These tensors represent pixel values, gradients, and intermediate results during the diffusion process. As you refine noise vectors and generate progressively clearer images, the VRAM acts as a temporary workspace. It stores these tensors, allowing for efficient data manipulation.
Intermediate Results:
Imagine you’re creating a mesmerizing digital landscape. Each diffusion step produces an intermediate result—a glimpse of the final image. The RTX 3060’s generous VRAM ensures that these snapshots are readily available, facilitating smooth transitions.
Model Parameters:
Stable Diffusion models consist of learnable parameters. These parameters guide the noise vector refinement. With 12 GB of VRAM, the RTX 3060 accommodates these parameters, enabling robust model training.
Gradients and Backpropagation:
During training, gradients flow backward through the neural network. The VRAM stores these gradients, allowing the model to adjust its weights. The RTX 3060’s ample memory ensures stable backpropagation, leading to better convergence.
Artistic Freedom and Creativity
Whether you’re an artist experimenting with abstract forms or a researcher exploring novel visual representations, VRAM capacity matters. Here’s why:
Canvas Size:
Larger VRAM allows for higher-resolution canvases. You can work on intricate details without worrying about memory constraints. Create expansive landscapes, intricate fractals, or dreamlike scenes—the RTX 3060 has your back.
Multitasking:
Perhaps you’re simultaneously running other creative software—3D modeling, video editing, or texture synthesis. The RTX 3060’s VRAM handles multitasking gracefully, ensuring a seamless workflow.
Future-Proofing:
As AI models evolve and demand more memory, having headroom is essential. The RTX 3060’s 12 GB provides a comfortable buffer for future innovations.
Making the Most of VRAM
Remember these tips:
Batch Size:
Experiment with batch sizes during training. Smaller batches fit comfortably in VRAM, while larger ones may require memory-efficient strategies.
Memory Optimization:
Optimize your code. Minimize unnecessary memory allocations and ensure efficient data movement.
3. Cost vs. Performance
While the RTX 3060 is a more budget-friendly option compared to higher-end RTX 3000 series cards like the RTX 3080 or 3090, it still delivers excellent performance in AI tasks, including Stable Diffusion. This balance of cost and performance makes it a popular choice for both AI enthusiasts and professionals alike.
Benchmarks and Real-World Performance
To validate our claims, let’s peek at some benchmarks and real-world scenarios:
Training Time:
When training Stable Diffusion models, the RTX 3060 demonstrates competitive performance. It converges swiftly, allowing you to iterate and experiment efficiently.
Image Generation:
Whether you’re creating dreamlike landscapes, abstract patterns, or realistic portraits, the RTX 3060 handles image generation with finesse. Its ability to maintain stability during diffusion steps ensures visually pleasing results.
Transfer Learning:
If you’re fine-tuning pre-trained models for Stable Diffusion, the RTX 3060 accelerates the process. Transfer learning benefits from its robust architecture.
When it comes to Stable Diffusion (SD), the battle between the NVIDIA GeForce RTX 3060 and the RTX 3070 revolves around two critical factors: VRAM capacity and speed. Let’s dive into the specifics, considering how these GPUs fare in the realm of AI-based image generation.
1. VRAM Matters, But Not Always
RTX 3060:
With its 12 GB of GDDR6 VRAM, the RTX 3060 provides a comfortable canvas for most SD tasks. When you’re working with large image tensors or handling intricate details, this VRAM capacity ensures stability.
RTX 3070:
Despite having 8 GB of VRAM, the 3070 compensates with its superior architecture and computational power. As long as you don’t push the VRAM limits, the 3070’s overall capabilities make it a worthy contender for SD.
2. The Need for Speed
RTX 3060:
While not as powerful as the 3070, the RTX 3060 still delivers commendable performance. Its clock speeds and CUDA cores contribute to efficient computations during SD tasks.
RTX 3070:
Here’s where the 3070 shines. Its raw computational power translates to faster processing. If speed is your priority, the 3070 takes the lead.
3. The Verdict
RTX 3060:
If you’re budget-conscious and prioritize a balance between VRAM and performance, the 3060 is a solid choice. It handles most SD scenarios without breaking a sweat.
RTX 3070:
For serious creators who demand efficiency, the 3070 is the go-to option. Its speed outweighs the VRAM difference, making it a formidable tool for SD.
Remember, the right GPU depends on your specific workflow and how close you dance to the VRAM edge. Whether you’re upscaling, transforming images, or exploring novel visual representations, choose wisely and let your creativity flow!

Is 8GB VRAM Enough for Stable Diffusion? It is a neural network technique for image generation and demands at least 8GB VRAM. Smaller images work smoothly, but larger canvases hunger for more memory. Optimized scripts and practical hacks enhance the experience.
Tips for Optimal Usage
To make the most of your RTX 3060:
Batch Size:
Experiment with batch sizes during training. Smaller batches may fit comfortably in the VRAM, while larger ones can exploit parallelism.
Regularization:
Apply regularization techniques to prevent overfitting. The RTX 3060’s computational power allows for efficient regularization.

In this guide, we will explore a hassle-free approach on How to Install Stable Diffusion, a powerful web user interface. What sets this method apart is the utilization of GitHub Desktop, which provides the unique advantage of easy rollback options. By leveraging GitHub Desktop, you gain the ability to effortlessly revert installations of both the…
Final Thoughts
In conclusion, the RTX 3060 is indeed a good choice for Stable Diffusion. It offers a great balance of performance, VRAM capacity, and cost, making it suitable for a wide range of AI image generation tasks. As with any tech purchase, ensure that it fits your specific needs and requirements before proceeding.
Stable Diffusion Hardware Requirements:
- System Requirements: Your Complete Guide
- Do you need a GPU for Stable Diffusion?
- What GPU is needed for Stable Diffusion?
- How much RAM do you need for Stable Diffusion?
- Is 2gb VRAM enough for Stable Diffusion?
- Is RTX 3060 good for Stable Diffusion?
- How many GPUs do you need to train Stable Diffusion?
- Is 8GB enough for Stable Diffusion? Is 8GB VRAM overkill?
- Do you need an SSD for Stable Diffusion?
- Can Stable Diffusion run on a CPU?
- Should I use CPU or GPU for Stable Diffusion?
Leave a Reply