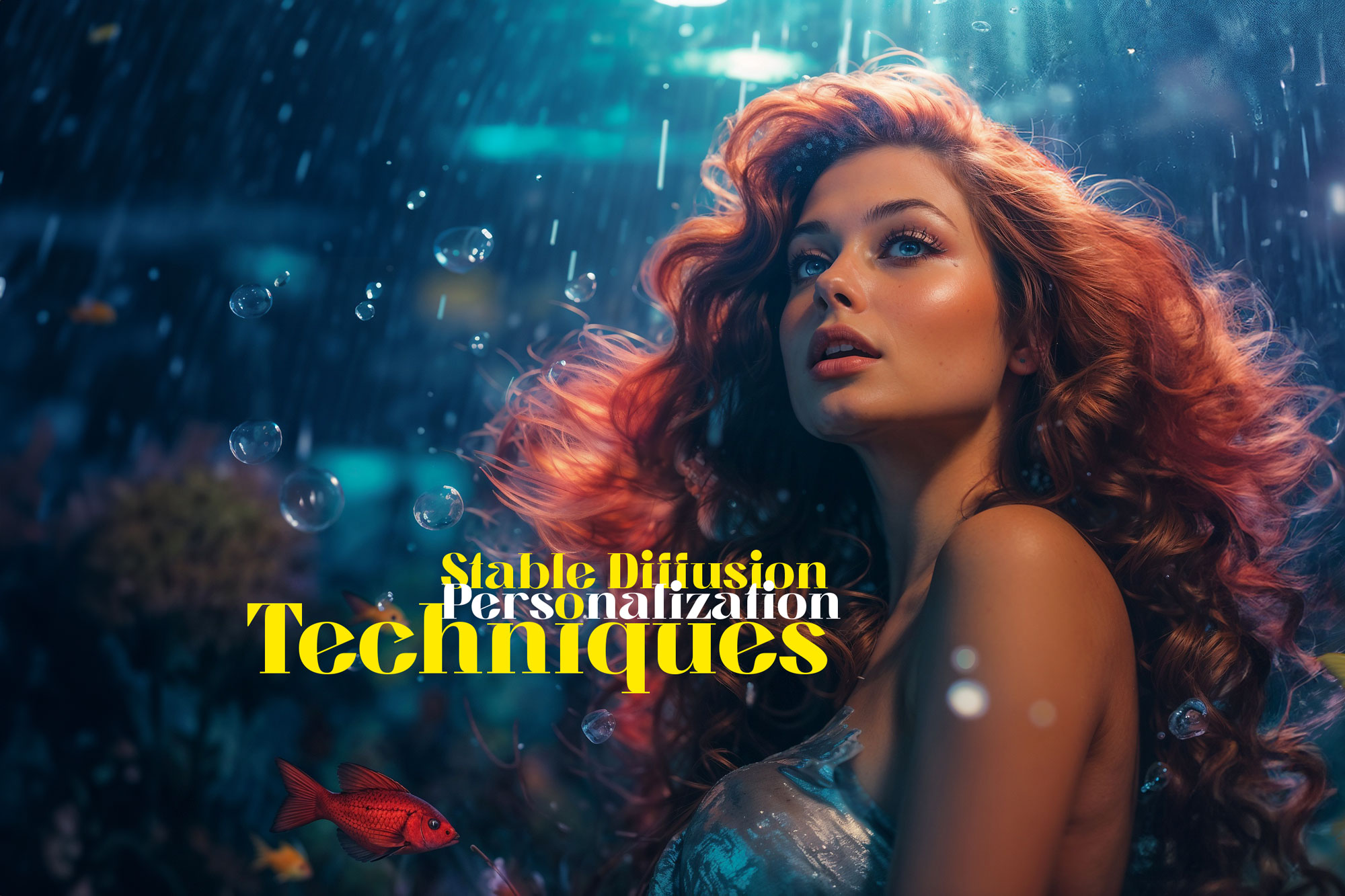
Stable Diffusion Personalization Techniques: Dreambooth vs LoRA vs Textual Inversion vs Hypernetworks
The ability to train custom models has become a vital skill across various fields. As AI continues to integrate into creative and technical domains, data annotation and model training are set to become valuable skill sets and some of the most sought-after professions. Within the creative field, we often use diffusion training, but when it comes to open-source localization, we have several options to choose from: Dreambooth vs LoRA vs Textual Inversion vs Hypernetworks. It can be a bit confusing, so which one should you use?
Table of Contents
The Personal Touch in AI Model Training
In the creative industry, AI is often seen as a double-edged sword—capable of imitating art but also accused of infringing on originality. However, the true potential of AI, especially with tools like Stable Diffusion, lies in its ability to personalize and not just plagiarize. Stable Diffusion, with its open-source nature, empowers you to train data locally, making it uniquely yours. You can train AI models with the paint strokes that are dictated by your hand, your style, your concepts. This is beyond copying; it’s more about creating something that’s authentically you and for you only.
Imagine training an AI model that knows your quirks, your preferences, and your signature style. It’s a collaborating agent that speaks your creative language. With methods like LoRa, Textual Inversion, Hypernetworks, and DreamBooth, you’re not just tweaking dials; you’re infusing your work with a piece of your soul. This is the future of the creative industry—a space where AI serves as an extension of the artist. By training your data, you’re setting the stage for AI that amplifies your skillsets turning you into a super soldier, but for artists.
Which method should you choose?
Whether it’s LoRa, DreamBooth, Textual Inversion, or Hypernetworks, each offers a unique path to personalizing your Stable Diffusion model. The decision hinges on what you want to achieve. Do you want to add a subtle twist to your work, or are you aiming for a bold transformation? Perhaps you’re looking to infuse your work with a specific character or style that’s all your own.
Dreambooth vs LoRA vs Textual Inversion vs Hypernetworks
Choosing the right method for you is like selecting the perfect brush in photoshop. This guide hopes to help guide you in the right direction in choosing the right method to get you started. To learn more, I will adapt each section with a guide to learning more about the training methods as I learn more about it.
LoRa (Low-Rank Adaptation)
LoRa modifies the attention mechanism of pre-trained models by introducing low-rank matrices. This allows for efficient fine-tuning with fewer parameters, making it resource-efficient. It’s particularly useful when you want to make quick adaptations to existing models without the need for extensive retraining or computational resources. However, its modifications are limited to the attention mechanism, which may not capture all desired nuances in the data. I use LoRa’s the most because it can be adapted and transported to any fine-tuned models, giving you a ton of flexibility to see what your trained content looks on other trained models.
Why choose LoRa?
- You have limited computational resources.
- You need quick adaptations to existing models.
- You’re focusing on efficiency over extensive model changes.
- You’re looking to adapt your trained LoRa’s on other existing models.
When to use LoRa: |
---|
Scenario: You have a pre-trained model and want to make small, incremental changes without retraining the entire model. |
Example: If you’re fine-tuning a model to recognize specific architectural styles, LoRa can quickly adapt the model to recognize new styles like Baroque or Gothic by adjusting the attention mechanism. |
Textual Inversion
Textual Inversion involves training on a small set of images to create new embeddings for specific concepts or styles. This method allows the model to generate images with new concepts not present in the original training data. It’s great for adding specific new concepts to the model without extensive retraining. The downside is that it can only learn a limited number of concepts from a small dataset.
Why choose Textual Inversion?
- You want to introduce new, specific concepts to the model.
- You have a small dataset of images for the new concept.
- You prefer not to alter the underlying model significantly.
When to use Textual Inversion: |
---|
Scenario: You need to introduce a new concept or term that doesn’t exist in the model’s vocabulary. |
Example: For an artist who wants to introduce their unique character, “Zephyra,” into the model, Textual Inversion can create an embedding that represents “Zephyra,” allowing the model to generate images of this character in various contexts. |
Hypernetworks
Hypernetworks are small neural networks that fine-tune the style of the model by modifying the cross-attention module. They enable fast training and have small file sizes, making them resource-efficient. Hypernetworks are suitable for rapid style adjustments with limited computational resources. However, they may not capture the full complexity of styles compared to larger models. I personally think that Hyprnetworks is an inferior version of LoRa’s, so I would not use it.
Why choose Hypernetworks?
- You’re aiming for rapid style adjustments.
- You have limited computational power.
- You prefer a method that’s resource-efficient and quick.
When to use Hypernetworks: |
---|
Scenario: You’re aiming for style transfer or want to apply a specific artistic filter to the images generated by the model. |
Example: A graphic designer wants to apply a “watercolor” effect to all generated images. Hypernetworks can be trained to modify the cross-attention module to apply this effect across different prompts. |
DreamBooth
DreamBooth fine-tunes diffusion models by injecting a custom subject into the model, allowing for personalized content creation. It enables the generation of images featuring specific real-life objects or individuals in various contexts. DreamBooth is VRAM intensive and potentially cost-prohibitive for hobbyist users, but it offers a high degree of personalization. This is what you really want to do if you want to train your own personalized model without any other data interference from unwanted training.
Why choose DreamBooth?
- You want to personalize models with specific subjects or styles.
- You have access to sufficient VRAM and computational resources.
- You’re looking for high-quality, personalized content generation.
When to use DreamBooth: |
---|
Scenario: You want to personalize the model with a specific subject, such as a person or a pet, and generate images of them in various settings. |
Example: If you want to generate images of your pet dog in fantasy landscapes, DreamBooth can train the model with pictures of your dog, allowing it to create personalized content. |
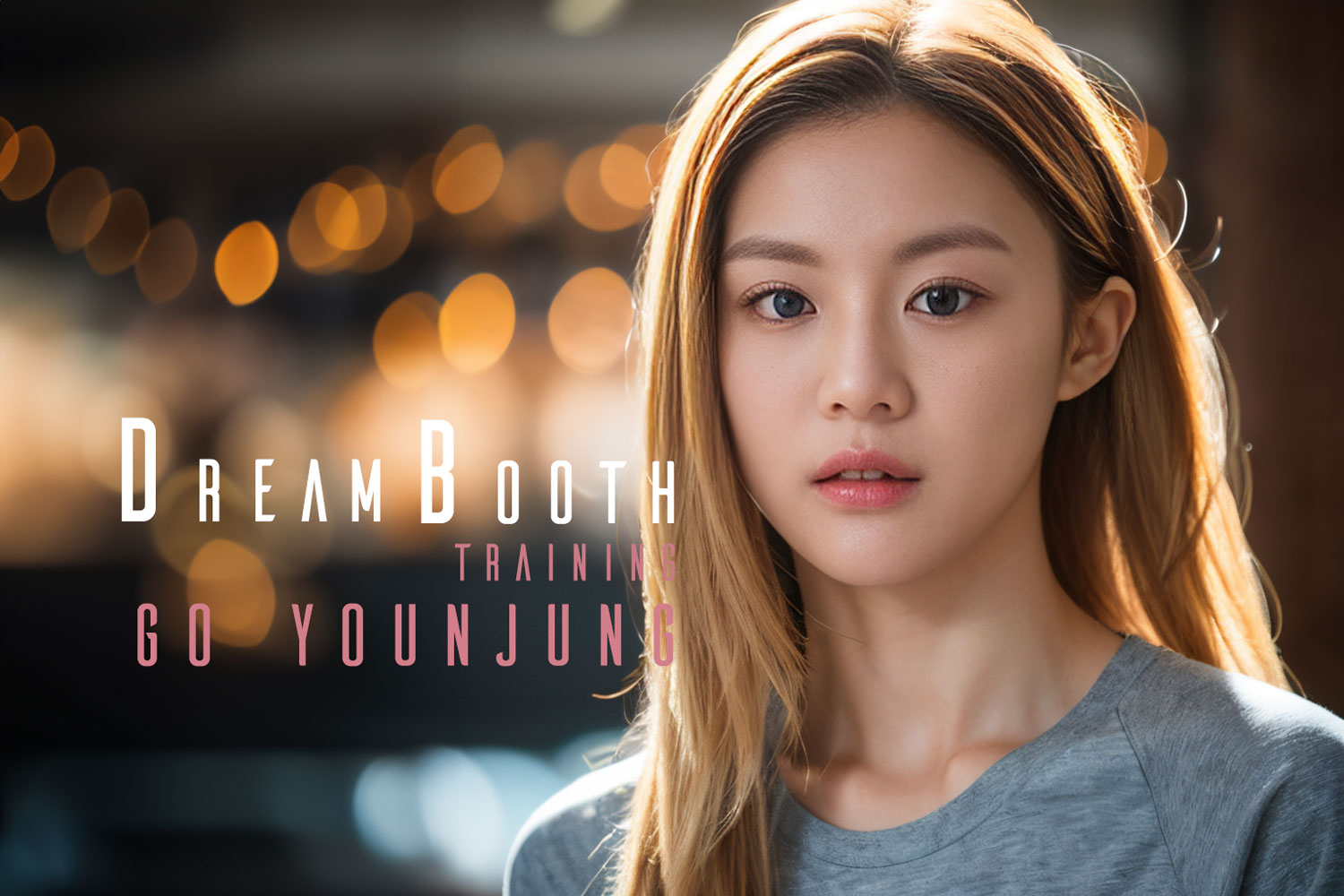
Inform and guide the learning process of Stable Diffusion by incorporating DreamBooth Captions. Improve image refinement and avoid unintended focus. Boost your results!
Strategic Application of Training Methods
Refining and extending the capabilities of a pre-existing model, such as one fine-tuned with DreamBooth, LoRa can be a great way to expand your repertoir. It allows for the creation of specific characters or styles without the need to retrain the entire model. This is like creating custom brushes in art software; just as artists create brushes in Photoshop or ZBrush to add unique textures or effects, LoRa can be used to create “brushes” for Stable Diffusion that apply particular traits or styles to generated images. It’s just on steroids.
By strategically selecting the appropriate method, you can maximize the effectiveness of your custom Stable Diffusion models, ensuring that they meet the specific needs of your creative projects.
Leave a Reply