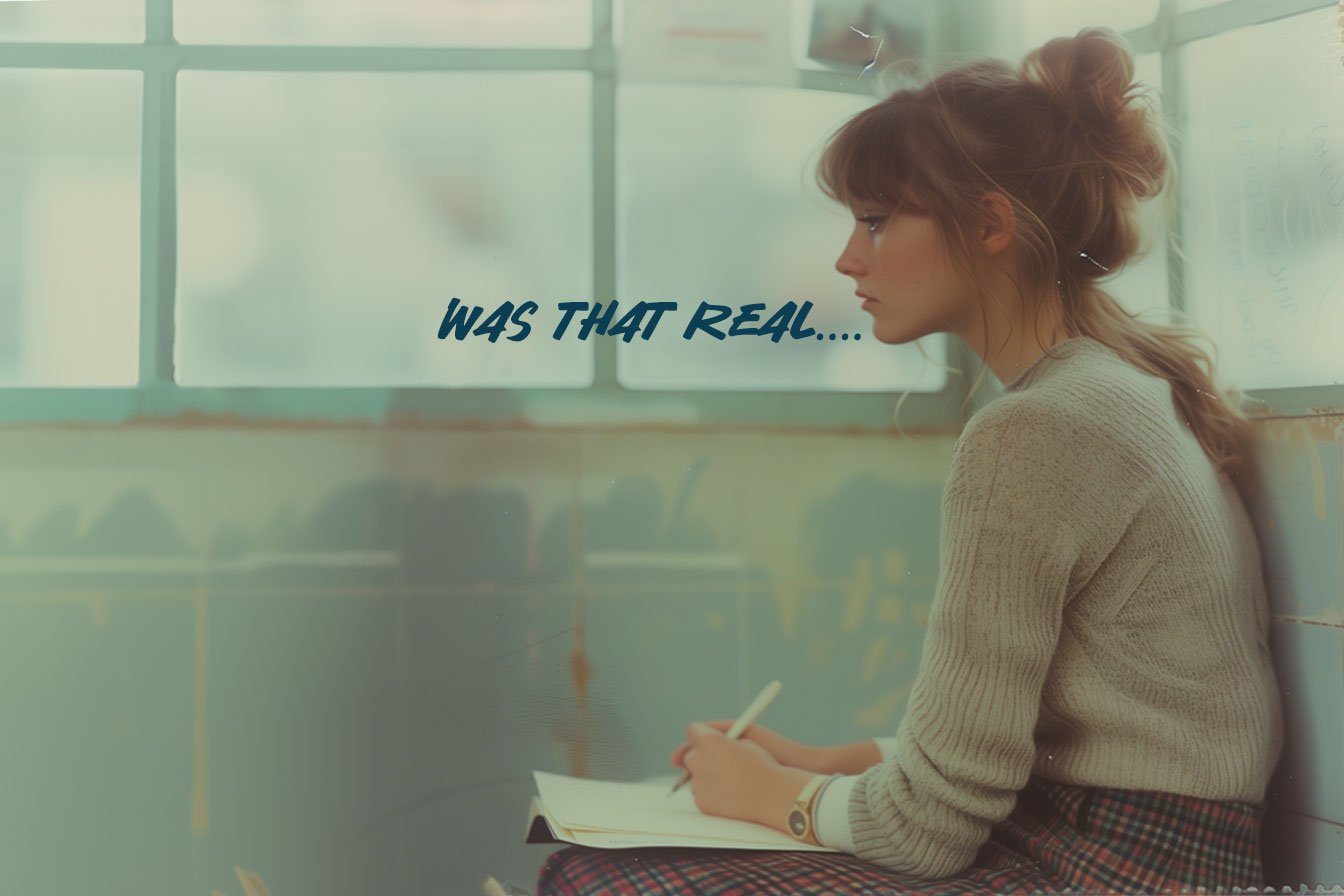
The Truth About How Stable Diffusion Works: Why It’s Not a Copycat
Stable Diffusion is a revolutionary technology that can generate realistic images from text descriptions. But how does it work? And is it really creating original art or just copying existing images? In this post, we’ll explore the science and the art behind how Stable Diffusion works and show you why it’s more than just a fancy tool. 🎨
Stable Diffusion is based on a type of deep generative model called latent diffusion model, which can learn to compress and decompress images in a latent space using noise. By combining this model with a text encoder, Stable Diffusion can condition the image generation on natural language inputs, allowing you to create any image you can imagine with just a few words.
Whether you’re an artist, a designer, a teacher, or a curious learner, Stable Diffusion can help you express your ideas, inspire your imagination, and have fun. But is Stable Diffusion real or fake? Is it actually creating new images or just reusing old ones? How does it add its own creativity and variation to the images? Let’s find out in this deep dive into the technology behind Stable Diffusion.
Table of Contents
What is Stable Diffusion and what can it do?
Stable Diffusion is a type of latent diffusion model, which is a kind of deep generative artificial neural network that can create photo-realistic images from text descriptions. You can try it online for free at Stable Diffusion Online or check out the open source code and model weights at GitHub. Stable Diffusion is fast, high-quality, and versatile, and can be used for various applications such as art, design, and education.
Stable Diffusion can generate images for any text prompt you can think of, such as “a blue cat with wings” or “a sunset over the ocean”. It can also create images in different styles, such as realistic, cartoon, or abstract. Here are some examples of Stable Diffusion outputs and how they match the text prompts:

Learn the ins and outs of Stable Diffusion Prompt Weights for Automatic1111. I’ll be sharing my findings, breaking down complex concepts into easy-to-understand language, and providing practical examples along the way.



A black and white portrait of Albert Einstein | A painting of a forest in the style of Van Gogh | A logo for a company called Stable Diffusion |
Stable Diffusion can also modify existing images using techniques such as inpainting, outpainting, and super-resolution. Inpainting is the process of filling in missing or damaged parts of an image, such as removing an unwanted object or restoring an old photo. Outpainting is the process of extending the boundaries of an image, such as adding more sky or landscape. Super-resolution is the process of increasing the quality and resolution of an image, such as enhancing a blurry or pixelated image. Here are some examples of how Stable Diffusion can transform images using these techniques:




Original Image | Inpainted Image | Outpainted Image | Super-Resolution Image |

Learn about Stable Diffusion Inpainting in Automatic1111! Explore the unique features, tools, and techniques for flawless image editing and content replacement.
As you can see, Stable Diffusion can do amazing things with images, both from text and from other images. But how does it work under the hood? How does it use noise and diffusion to create realistic and diverse images? Let’s find out in the next section.
How Stable Diffusion works under the hood?
Stable Diffusion is based on a type of deep generative model called latent diffusion model, which can learn to compress and decompress images in a latent space using noise . A latent space is a high-dimensional vector space that represents the essential features and variations of the data. By mapping images to and from the latent space, Stable Diffusion can generate new images that are similar but not identical to the original ones.
The main components of Stable Diffusion are the autoencoder, the U-Net, and the text encoder. The autoencoder is a neural network that consists of two parts: the encoder and the decoder. The encoder takes an image as input and transforms it into a latent vector, which is a compact representation of the image. The decoder takes a latent vector as input and reconstructs the image as output.
The U-Net is a special type of autoencoder that has skip connections between the encoder and the decoder, which allows it to preserve more details and spatial information of the image. The text encoder is another neural network that takes a text prompt as input and converts it into a text vector, which is a semantic representation of the text.
Stable Diffusion uses a process called diffusion to compress and decompress images in the latent space. Diffusion is the movement of particles from regions of high concentration to regions of low concentration. In Stable Diffusion, diffusion is applied to the pixels of the image, which are treated as particles. The diffusion process has two phases: the forward phase and the reverse phase.
In the forward phase, Stable Diffusion adds noise to the image gradually, until it becomes completely noisy and unrecognizable. This is like diffusing the pixels of the image until they are evenly distributed. The noise level is controlled by a parameter called the noise schedule, which determines how much noise is added at each step. The noise schedule is learned by the model during training. The forward phase can be seen as a compression process, as it reduces the information of the image to the noise schedule and the latent vector.
In the reverse phase, Stable Diffusion removes noise from the image gradually, until it becomes clear and realistic. This is like reversing the diffusion process and restoring the pixels of the image. The reverse phase can be seen as a decompression process, as it reconstructs the image from the noise schedule and the latent vector.
However, the reverse phase is not a simple inverse of the forward phase, as it also uses the text vector to condition the image generation on the text prompt. The text vector is combined with the latent vector at each step, and the U-Net uses both vectors to predict the next image. This way, the U-Net can generate images that match the text prompt and the style of the original image.
Here are some examples of how Stable Diffusion compresses and decompresses images using noise and diffusion:
The Math From Scratch
Source by Sergios Karagiannakos and Nikolas Adaloglou

As you can see, Stable Diffusion can create realistic and diverse images from text descriptions using noise and diffusion. But is Stable Diffusion real or fake? Is it actually creating new images or just reusing old ones? How does it add its own creativity and variation to the images? Let’s find out in the next section.
Is Stable Diffusion Real or Fake?
Stable Diffusion can create realistic and diverse images from text descriptions using noise and diffusion. But is Stable Diffusion real or fake? Is it actually creating new images or just reusing old ones? How does it add its own creativity and variation to the images?
Some people might think that Stable Diffusion is just copying and pasting existing images from the internet, or that it is not really generating art, but just mimicking human artists. However, these claims are not true. Stable Diffusion is not a copycat, but a creative and versatile tool that can help you express your ideas, inspire your imagination, and have fun.
First of all, Stable Diffusion does not memorize or reproduce the images that it was trained on. It only learns the general features and patterns of the images, such as shapes, colors, textures, and styles. It does not store or access the exact images that it saw during training. Therefore, it cannot copy and paste any image that you give it as a text prompt.
Secondly, Stable Diffusion does not always generate the same image for the same text prompt. It adds its own randomness and variation to the image generation process, which makes the results more diverse and interesting.
For example, if you give it the text prompt “a cat wearing a hat”, it can generate different images of cats wearing different hats, with different poses, expressions, and backgrounds. You can also change the random seed, which is a number that influences the randomness of the image generation, to get different results for the same text prompt.
Thirdly, Stable Diffusion can generate images that are novel, original, and sometimes surprising. It can create images that do not exist in the real world, such as mythical creatures, fantasy landscapes, or futuristic inventions. It can also create images that are unexpected, humorous, or absurd, such as a banana with a face, a dog driving a car, or a pineapple wearing sunglasses. These images can challenge your perception, spark your curiosity, and make you laugh. Give it a try for free. You can learn how to install it locally on your computer below.
As you can see, Stable Diffusion is a fascinating technology that combines deep learning and diffusion to create stunning images from text. It’s not just a copycat, but a creative and versatile tool that can help you express your ideas, inspire your imagination, and have fun. Have you tried Stable Diffusion yet? What kind of images did you create? Let us know in the comments below!

This is the Stable Diffusion prerequisite guide. Here we will learn how to prepare your system for the installation of Stable Diffusion’s distinct Web UIs—Automatic1111, Invoke 3.0, and Comfy UI
Understanding Stable Diffusion:
- What is Stable Diffusion?
- Is Stable Diffusion real?
- What do steps do in Stable Diffusion?
- What is the latest Stable Diffusion?
- How many people use Stable Diffusion?
- Is Stable Diffusion easy to use?
- What is similar to Stable Diffusion?
- What is Stable Diffusion style?
- How does Stable Diffusion training work?
- Is Stable Diffusion Pretrained?
- Can Stable Diffusion generate 3D models?
- How do you use Stable Diffusion at home?
- What is the new version of Stable Diffusion?
- What are the applications of Stable Diffusion?
Leave a Reply