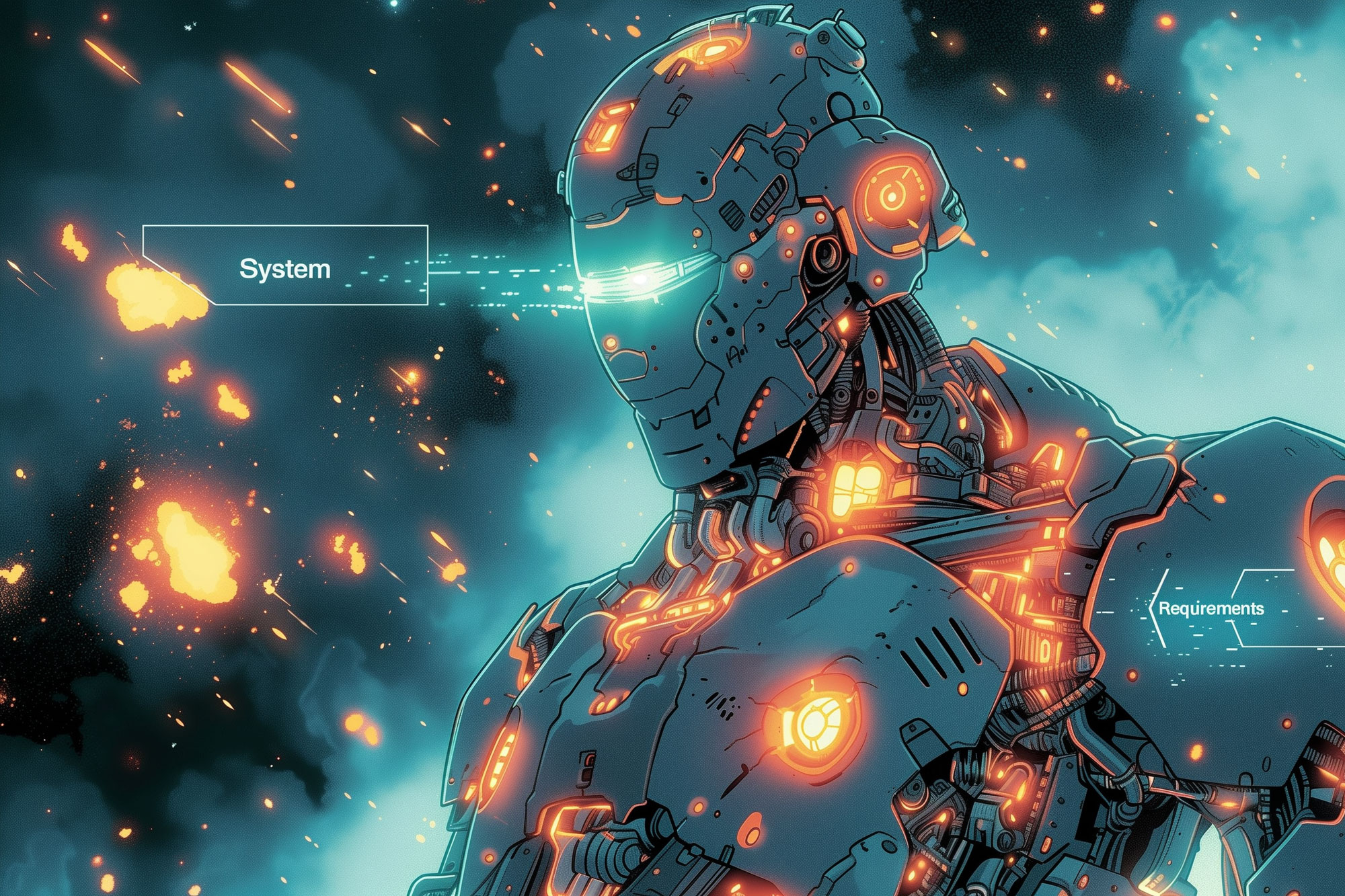
System Requirements for Stable Diffusion: Your Complete Guide
Stable Diffusion, one of the most popular AI art-generation tools, offers impressive results but demands a robust system. Whether you’re a creative artist or an enthusiast, understanding the System Requirements for Stable Diffusion is important for efficient and smooth operation. In this comprehensive guide, we’ll go deep into the specifics of running Stable Diffusion effectively, from low-budget setups to high-end configurations.

Table of Contents
Stable Diffusion is a cutting-edge artificial intelligence model that specializes in generating detailed and creative images based on textual descriptions. This transformative technology harnesses the power of deep learning to interpret text inputs and produce visually compelling outputs, bridging the gap between human creativity and machine efficiency. At its core, Stable Diffusion operates through a complex interplay of algorithms that analyze the given text, understand the context and visual elements described, and then generate an image that matches the description as closely as possible.
How It Operates
The operation of Stable Diffusion is rooted in a type of neural network architecture known as a Latent Diffusion Model. This model works by gradually refining random noise into a coherent image through a series of steps, guided by the textual description it is given. The process involves encoding the text into a format that the model can understand, then iteratively adjusting the initially random image until it aligns with the encoded text description. This iterative refinement is what allows Stable Diffusion to create images that are both intricate and aligned with the user’s intent.
The technology behind Stable Diffusion is built upon a vast dataset of images and their descriptions, allowing the model to learn a wide range of styles, objects, and scenes. As a result, it can generate anything from realistic portraits and landscapes to fantastical creatures and abstract art, making it an incredibly versatile tool for creators.
Importance of System Requirements for Efficient Operation
The sophistication of Stable Diffusion’s operation means that it requires significant computational resources to function effectively. The model’s ability to process and generate high-quality images is heavily dependent on the hardware it runs on. Key factors include the processing power of the CPU, the capabilities of the GPU (especially important for handling the intensive computations of deep learning models), and the amount of RAM and VRAM available to store and manage the model’s data during operation.
Efficient operation of Stable Diffusion is crucial for several reasons:
- Speed: Higher-end systems can generate images more quickly, reducing wait times from minutes to seconds, which is vital for iterative creative processes.
- Quality: More powerful systems can handle higher-resolution generations, allowing for more detailed and complex images.
- Stability: Adequate system resources ensure that the software runs smoothly without crashing, especially when processing large batches of images or running for extended periods.
Understanding and meeting the system requirements for Stable Diffusion is therefore essential for anyone looking to explore the full potential of this AI art generation tool. Whether you’re working on a personal project or a professional assignment, the right hardware setup can significantly impact the quality of your results and your overall experience with the technology.

To effectively run Stable Diffusion, it’s very important to understand the hardware specifications that will impact its performance. Below, we explain the key components involved and provide a general overview of the minimum system requirements needed to run Stable Diffusion. These requirements are a baseline for getting started, though higher specifications will significantly enhance performance and the overall experience.
Key Hardware Components
- CPU (Central Processing Unit): Acts as the brain of your computer, executing instructions from software applications. For Stable Diffusion, a capable CPU can speed up preprocessing steps and manage tasks not offloaded to the GPU.
- GPU (Graphics Processing Unit): Specialized for parallel processing, GPUs are critical for deep learning tasks, including running models like Stable Diffusion. They significantly accelerate image generation.
- RAM (Random Access Memory): Temporary storage that your computer uses to hold data that is frequently accessed by the CPU. Adequate RAM allows for smooth operation of software applications and the handling of large models.
- VRAM (Video RAM): Dedicated memory used by the GPU to store images, textures, and other graphics data. Higher VRAM is essential for generating high-resolution images with Stable Diffusion.
General Minimum Requirements
The minimum system requirements for running Stable Diffusion effectively can vary based on the specific version or fork of the model you are using. However, the following table provides a general guideline for the baseline hardware needed to get started:
Component | Minimum Requirement |
---|---|
CPU | Quad-core processor (Intel i5/i7 series or AMD Ryzen equivalent) |
GPU | NVIDIA GTX 1060 6GB or AMD equivalent with at least 6GB VRAM |
RAM | 8GB |
VRAM | 6GB |
Storage | SSD with at least 20GB of free space |
OS | Windows 10, Linux (Ubuntu 18.04 or later), or macOS (with limitations) |
Notes:
- These specifications are the minimum for running Stable Diffusion at basic levels of performance. For higher resolutions or faster generation times, more powerful hardware is recommended.
- Some forks or versions of Stable Diffusion may have specific requirements or optimizations that allow them to run on lower-end hardware or more efficiently utilize higher-end hardware.
- Running Stable Diffusion without a GPU is possible but significantly slower, making a compatible NVIDIA or AMD GPU a crucial component for practical use.
- Users should also consider the software requirements, such as Python and necessary libraries, which may have their own requirements for efficient operation.
Remember, these guidelines serve as a starting point. Depending on your specific use case, such as generating high-resolution art, running multiple instances, or experimenting with different models, you may need to exceed these minimum specifications.
To provide a more detailed and helpful guide, especially considering the nuances of GPU performance and the specific requirements for Stable Diffusion, let’s revisit the tables for low-end, mid-range, and high-end specifications. This time, we’ll include a more comprehensive list of popular graphics cards for each category, considering their VRAM, which is a critical factor for Stable Diffusion performance.
Low-End Specs
Ideal for beginners or those with a limited budget. These systems can run Stable Diffusion but may have limitations in terms of speed and output quality.
Component | Minimum Requirement |
---|---|
CPU | Quad-core processor (e.g., Intel i5 or AMD Ryzen 3) |
GPU | – NVIDIA GTX 1050 Ti (4GB VRAM) |
– NVIDIA GTX 1060 (6GB VRAM) | |
– NVIDIA GTX 1080 (8GB VRAM) – offers better performance within the low-end range | |
– AMD RX 570 (4GB VRAM) | |
– AMD RX 580 (8GB VRAM) | |
RAM | 8GB |
VRAM | 4GB minimum, 6-8GB for better performance |
Storage | 50GB HDD or SSD |
Expected Performance and Limitations:
- Slower generation times, especially for higher resolutions.
- May require lower resolution or complexity in generated images.
- Suitable for basic use and experimentation.
Mid-Range Specs
Designed for enthusiasts and semi-professional users who seek a good balance between cost and performance.
Component | Recommended Specifications |
---|---|
CPU | 6-core processor (e.g., Intel i7 or AMD Ryzen 5) |
GPU | – NVIDIA RTX 2060 (6GB VRAM) |
– NVIDIA RTX 3060 (12GB VRAM) – note the higher VRAM compared to some higher-end models | |
– NVIDIA GTX 1080 Ti (11GB VRAM) | |
– AMD RX 5600 XT (6GB VRAM) | |
– AMD RX 5700 (8GB VRAM) | |
RAM | 16GB |
VRAM | 6GB minimum, 8-12GB for improved performance |
Storage | 256GB SSD |
Performance Improvements Over Low-End Specs:
- Faster image generation with improved resolution.
- Capable of handling more complex tasks with better efficiency.
High-End Specs
For professional use, offering the fastest performance and highest quality outputs.
Component | High-Performance Specifications |
---|---|
CPU | 8-core processor or higher (e.g., Intel i9 or AMD Ryzen 7/9) |
GPU | – NVIDIA RTX 3070 (8GB VRAM) |
– NVIDIA RTX 3080 (10GB VRAM) | |
– NVIDIA RTX 3090 (24GB VRAM) – top-of-the-line performance | |
– AMD RX 6800 XT (16GB VRAM) | |
– AMD RX 6900 XT (16GB VRAM) | |
RAM | 32GB or more |
VRAM | 8GB minimum, 10-24GB for the best performance |
Storage | 1TB NVMe SSD |
Benefits of High-End Specs:
- Significantly faster generation times, suitable for high-resolution and complex image generation.
- Enhanced ability to run multiple instances or handle intensive tasks simultaneously.
Including a variety of popular GPUs in each category clarifies the options available to users based on their budget and performance needs. Notably, the NVIDIA RTX 3060’s higher VRAM makes it an attractive option for users needing more memory for tasks like Stable Diffusion, despite being in the mid-range category. This nuanced understanding helps in making more informed decisions when planning or upgrading your system for Stable Diffusion.
To run Stable Diffusion effectively, aside from having the necessary hardware, specific software and library requirements must be met. This section covers the operating system compatibility and essential software components, including programming languages, libraries, and development tools needed for Stable Diffusion.
Operating System Compatibility
- Windows: Stable Diffusion is compatible with Windows 10 and newer versions. Windows offers an accessible platform for running various forks and versions of Stable Diffusion, with broad support for necessary software and drivers.
- Linux: Many users prefer Linux for running Stable Diffusion due to its robustness and the control it offers. Popular distributions like Ubuntu 18.04 LTS or newer are well-supported. Linux environments often yield better performance and resource management.
- macOS: Running Stable Diffusion on macOS is possible, but with limitations. Due to the lack of NVIDIA GPU support (for CUDA), running the model might require workarounds, such as using external GPUs or relying on CPU-only modes, which are significantly slower.
Necessary Software and Libraries
- Python: Stable Diffusion is primarily developed in Python, requiring Python 3.8 or newer. Python serves as the foundation for running the scripts and managing other library dependencies.
- PyTorch: A key library for running Stable Diffusion, PyTorch provides the deep learning framework necessary for the model. Installation should be compatible with the version that supports your GPU (CUDA-enabled for NVIDIA GPUs).
- CUDA: For NVIDIA GPU users, CUDA (Compute Unified Device Architecture) is essential for leveraging GPU acceleration. CUDA allows PyTorch and Stable Diffusion to run computations on the GPU, significantly speeding up image generation. The required version depends on your GPU and the version of PyTorch installed but starting with CUDA 11.x is commonly recommended.
- cuDNN: NVIDIA’s CUDA Deep Neural Network library (cuDNN) is a GPU-accelerated library for deep learning that optimizes some operations for neural network training and inference. It’s essential for achieving optimal performance on NVIDIA hardware.
- Visual Studio Code (VS Code) or other IDEs: While not mandatory, having an Integrated Development Environment (IDE) like Visual Studio Code can simplify the process of editing scripts, managing projects, and debugging. For Windows users, installing Visual Studio with C++ support is also recommended to ensure compatibility with various Python libraries that require compilation.
- Additional Python Libraries: Running Stable Diffusion requires various Python libraries, which can be installed via pip. Key libraries include:
- torchvision: For image processing tasks complementary to PyTorch.
- numpy: Adds support for large, multi-dimensional arrays and matrices, along with a large collection of high-level mathematical functions to operate on these arrays.
- Pillow (PIL): An imaging library required for opening, manipulating, and saving many different image file formats.
- Transformers: Provides thousands of pre-trained models to perform tasks on texts such as classification, information extraction, question answering, summarization, translation, text generation, etc., in over 100 languages.
- Git: Useful for cloning the Stable Diffusion repository or any of its forks from GitHub.
These software and library requirements are critical for the successful operation of Stable Diffusion, ensuring that the system is capable of executing the model’s code, managing dependencies, and efficiently performing computations. Before installation, verify the compatibility of these components with your hardware and operating system to avoid conflicts and ensure optimal performance.

This is the Stable Diffusion prerequisite guide. Here we will learn how to prepare your system for the installation of Stable Diffusion’s distinct Web UIs—Automatic1111, Invoke 3.0, and Comfy UI
The versatility of Stable Diffusion has led to the development of various forks and user interfaces (UIs), each designed to enhance usability and offer unique features. Below, we explore some of the most popular options: Automatic1111, WebUI Forge, InvokeAI, and ComfyUI. Understanding their system requirements and unique offerings can help you choose the best fit for your needs and system specifications.
Automatic1111’s WebUI
Overview: Automatic1111’s WebUI is one of the most popular and comprehensive interfaces for Stable Diffusion, known for its extensive feature set and user-friendly design. It includes support for text-to-image, image-to-image, inpainting, outpainting, and more.
System Requirements: Runs on Windows, Linux, and macOS (with limitations due to GPU support). It requires a compatible GPU with a minimum of 4GB VRAM, although 6GB or more is recommended for optimal performance. Python, PyTorch, and CUDA (for NVIDIA GPUs) are essential.
Unique Features: Offers an intuitive web-based interface, batch processing, a wide range of models, and plugins for additional functionality.

Installing the Automatic1111 Web UI for Stable Diffusion requires a solid groundwork. If you’ve been following our guide series, you’ve likely laid down this essential foundation. This tutorial builds upon the preparatory steps detailed in our previous blog.
WebUI Forge
Overview: WebUI Forge is a fork focused on enhancing the user experience with improvements in performance and ease of use. It builds on Automatic1111’s WebUI, aiming to streamline operations and add new features.
System Requirements: Similar to Automatic1111, it requires a decent GPU with adequate VRAM, Python, PyTorch, and CUDA for NVIDIA users. It’s designed to run on Windows and Linux.
Unique Features: Includes optimizations for faster processing, an improved user interface, and additional customization options not found in the original WebUI.
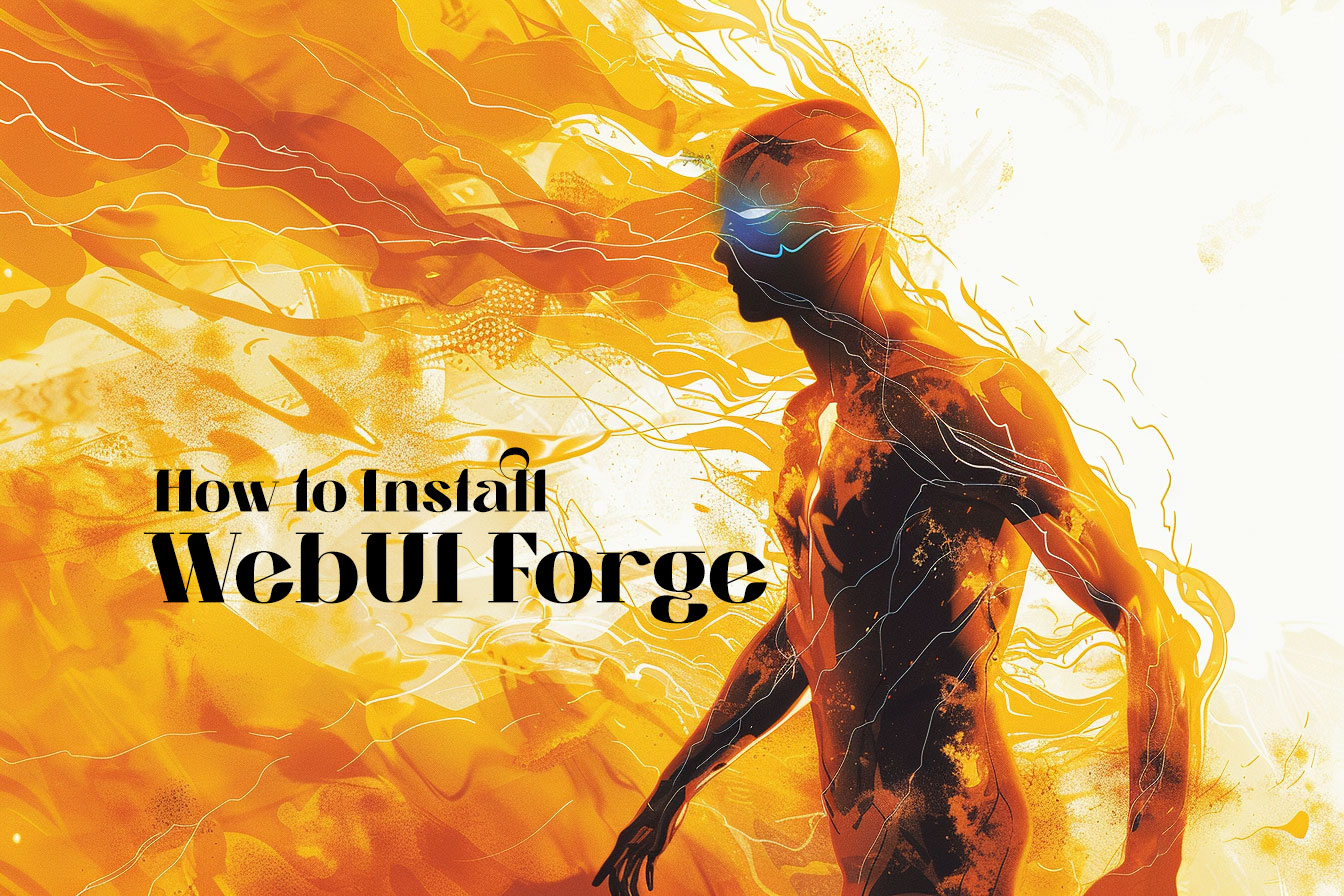
Installing the WebUI Forge for Stable Diffusion requires a solid groundwork. If you’ve been following our guide series, you’ve likely laid down this essential foundation. This tutorial builds upon the preparatory steps detailed in our previous blog so that you can learn how to Install WebUI Forge for Stable Diffusion.
InvokeAI
Overview: InvokeAI (formerly known as LATENT Diffusion) offers a user-friendly approach to interacting with Stable Diffusion, with a focus on simplicity and ease of use for beginners.
System Requirements: Compatible with Windows, Linux, and macOS. It can run on lower-end GPUs but performs best with a GPU that has at least 4GB of VRAM. Dependencies include Python and PyTorch, with CUDA recommended for NVIDIA GPU users.
Unique Features: Simplified setup process, clean interface, and support for various Stable Diffusion models. It’s geared towards users looking for a straightforward experience.

In this tutorial, you will learn how to install Invoke for Stable Diffusion and how to use it to generate amazing images from your own prompts.
ComfyUI
Overview: ComfyUI is a newer entry that emphasizes a comfortable and accessible user experience, making Stable Diffusion approachable for non-technical users.
System Requirements: The requirements are in line with other forks, needing a capable GPU, Python, and PyTorch. It’s designed to be lightweight, offering good performance even on mid-range systems.
Unique Features: Features a minimalistic and easy-to-navigate interface, with support for basic and advanced functionalities of Stable Diffusion. It focuses on removing complexity from the user’s perspective.

In this guide, we will show you how to install ComfyUI and use it to create stunning generative art with Stable Diffusion. ComfyUI is a powerful and flexible web UI that lets you create realistic images from text or other images using Stable Diffusion, a state-of-the-art technique for image synthesis. ComfyUI has many advantages over other Stable Diffusion GUIs, such as Automatic1111.
Fooocus
Overview: Fooocus is another notable interface for Stable Diffusion, aimed at streamlining the user experience and providing efficient image generation capabilities. It focuses on performance and ease of use, catering to both casual users and more demanding creative professionals.
System Requirements: Similar to other interfaces, Fooocus works best with a dedicated GPU (with at least 4GB VRAM recommended), Python, PyTorch, and, for optimal performance, CUDA for NVIDIA GPU users. It is compatible with Windows, Linux, and macOS, though macOS users may face the usual limitations related to GPU support.
Unique Features: Fooocus distinguishes itself with a clean, straightforward UI designed to minimize distractions and enhance productivity. It integrates well with various Stable Diffusion models and offers features such as quick access to presets, efficient management of generation tasks, and tools designed to streamline the creative workflow.
Choosing the Right Fork/UI
When selecting a fork or UI for Stable Diffusion, consider the following:
- System Specifications: Match the fork’s requirements with your hardware. Users with high-end GPUs and ample VRAM may opt for more feature-rich forks like Automatic1111 or WebUI Forge, while those with modest setups might prefer InvokeAI or ComfyUI.
- Ease of Use: Beginners may lean towards forks with simpler interfaces and setup processes, such as InvokeAI or ComfyUI.
- Features and Flexibility: Evaluate the features important to you, whether it’s batch processing, plugins, or support for various models. More advanced users might prioritize flexibility and customization options.
- Community and Support: Larger communities often mean better support and more frequent updates. Consider the community behind each fork for troubleshooting and enhancements.
By aligning your system’s capabilities and your personal needs with the features offered by these forks and UIs, you can choose the most suitable interface to enhance your Stable Diffusion experience.
Optimizing Stable Diffusion on various systems involves a combination of hardware upgrades, software adjustments, and configuration tweaks to maximize performance and efficiency. While specific optimization strategies may vary depending on the hardware and software environment, there are general tips that can help improve the operation of Stable Diffusion across most setups. Below are some guidelines and optimizations drawn from community recommendations and the official GitHub wiki, where applicable.
Optimizing Stable Diffusion on various systems involves a combination of hardware upgrades, software adjustments, and configuration tweaks to maximize performance and efficiency. While specific optimization strategies may vary depending on the hardware and software environment, there are general tips that can help improve the operation of Stable Diffusion across most setups. Below are some guidelines and optimizations drawn from community recommendations and the official GitHub wiki, where applicable.
- Upgrade GPU: The most impactful hardware upgrade for improving Stable Diffusion performance is a more powerful GPU, especially one with higher VRAM. NVIDIA GPUs benefit from CUDA acceleration, which significantly speeds up deep learning tasks.
- Increase RAM: More system memory (RAM) allows for larger models to be loaded and can improve overall system responsiveness, especially when multitasking.
- Use SSDs: Running Stable Diffusion on a system with an SSD (Solid State Drive) rather than an HDD (Hard Disk Drive) can reduce load times and improve the speed of model loading and image saving.
Software and Configuration Optimizations
- Adjust Batch Sizes: Depending on your system’s VRAM, adjusting the batch size for image generation can improve efficiency. Smaller batch sizes require less VRAM, while larger batches can speed up processing time if your GPU has the capacity.
- Use Efficient Models: Some forks of Stable Diffusion offer optimized models that require less computational power for similar or slightly reduced quality outputs. These can be particularly useful for lower-end systems.
- Optimize PyTorch and CUDA Versions: Ensure you’re using the most compatible version of PyTorch and CUDA for your GPU. Mismatches can lead to suboptimal performance.
- Enable Mixed Precision: If supported by your hardware, enabling mixed precision (using both 16-bit and 32-bit floating-point arithmetic in computations) can significantly speed up model inference times while maintaining image quality.
Tips from the Official Optimizations Page
- Model Pruning: Reducing the size of the model by pruning less important parameters can decrease the computational load, allowing for faster generation times.
- FP16 Inference: Utilizing FP16 (16-bit floating-point) inference where supported can nearly double the speed of image generation compared to using FP32, with minimal impact on output quality.
- Optimize Inference Code: Some forks and versions of Stable Diffusion have optimized inference code that can run faster on specific hardware setups. Reviewing the documentation and community forums for these optimizations can yield significant performance improvements.
- Parallel Processing: If you have a CPU with many cores, or multiple GPUs, some versions of Stable Diffusion support parallel processing, distributing the workload across available resources to speed up image generation.
Additional Considerations
- Cooling Solutions: Effective cooling can prevent thermal throttling, especially in high-end GPUs under heavy load, maintaining consistent performance.
- Software Updates: Keeping your operating system, drivers (especially GPU drivers), and Stable Diffusion forks up to date can ensure compatibility and often performance improvements.
- Community Resources: The Stable Diffusion community is constantly finding and sharing new optimizations. Engaging with community forums and GitHub discussions can provide access to the latest tweaks and tips.
Implementing these optimizations requires a balance between hardware capabilities, software configurations, and the specific needs of your projects. Starting with software and configuration tweaks is cost-effective and can provide immediate improvements, while hardware upgrades are a longer-term investment for significant performance boosts.
Incorporating command-line argument optimizations can significantly enhance the performance of Stable Diffusion, especially when using interfaces like AUTOMATIC1111’s WebUI, which supports a wide range of customizable settings. These optimizations are designed to fine-tune the model’s operation, allowing for faster image generation and more efficient resource usage on various systems. Here’s an updated guide integrating these command-line argument optimizations, with reference to the suggestions found on the AUTOMATIC1111 stable-diffusion-webui optimizations page.
Enhanced Optimizations Guide for Automatic1111 and WebUI Forge
Command-line Argument | Explanation |
---|---|
–opt-sdp-attention | May result in faster speeds than using xFormers on some systems but requires more VRAM. (non-deterministic) |
–opt-sdp-no-mem-attention | May result in faster speeds than using xFormers on some systems but requires more VRAM. (deterministic, slightly slower than –opt-sdp-attention and uses more VRAM) |
–xformers | Use xFormers library. Great improvement to memory consumption and speed. Nvidia GPUs only. (deterministic as of 0.0.19 [webui uses 0.0.20 as of 1.4.0]) |
–force-enable-xformers | Enables xFormers regardless of whether the program thinks you can run it or not. Do not report bugs you get running this. |
–opt-split-attention | Cross attention layer optimization significantly reducing memory use for almost no cost (some report improved performance with it). Black magic. On by default for torch.cuda, which includes both NVidia and AMD cards. |
–disable-opt-split-attention | Disables the optimization above. |
–opt-sub-quad-attention | Sub-quadratic attention, a memory efficient Cross Attention layer optimization that can significantly reduce required memory, sometimes at a slight performance cost. Recommended if getting poor performance or failed generations with a hardware/software configuration that xFormers doesn’t work for. On macOS, this will also allow for generation of larger images. |
–opt-split-attention-v1 | Uses an older version of the optimization above that is not as memory hungry (it will use less VRAM, but will be more limiting in the maximum size of pictures you can make). |
–medvram | Makes the Stable Diffusion model consume less VRAM by splitting it into three parts – cond (for transforming text into numerical representation), first_stage (for converting a picture into latent space and back), and unet (for actual denoising of latent space) and making it so that only one is in VRAM at all times, sending others to CPU RAM. Lowers performance, but only by a bit – except if live previews are enabled. |
–lowvram | An even more thorough optimization of the above, splitting unet into many modules, and only one module is kept in VRAM. Devastating for performance. |
*do-not-batch-cond-uncond | Only before 1.6.0: prevents batching of positive and negative prompts during sampling, which essentially lets you run at 0.5 batch size, saving a lot of memory. Decreases performance. Not a command line option, but an optimization implicitly enabled by using –medvram or –lowvram. In 1.6.0, this optimization is not enabled by any command line flags, and is instead enabled by default. It can be disabled in settings, Batch cond/uncond option in Optimizations category. |
–always-batch-cond-uncond | Only before 1.6.0: disables the optimization above. Only makes sense together with –medvram or –lowvram. In 1.6.0, this command line flag does nothing. |
–opt-channelslast | Changes torch memory type for stable diffusion to channels last. Effects not closely studied. |
–upcast-sampling | For Nvidia and AMD cards normally forced to run with –no-half, should improve generation speed. |
Adjusting for System Capabilities
- Batch Sizes: Smaller batch sizes reduce VRAM consumption, allowing Stable Diffusion to run on systems with less powerful GPUs. Conversely, larger batch sizes on more capable systems can improve throughput.
- Model Pruning and Efficient Models: As mentioned, utilizing pruned or efficiency-optimized versions of Stable Diffusion models can yield significant performance gains on all types of systems.
Software and Configuration Tweaks
- PyTorch and CUDA Compatibility: Ensure the versions of PyTorch and CUDA installed are fully compatible with your GPU, as this can affect both performance and stability.
- Mixed Precision (AMP): Where supported, activating Automatic Mixed Precision can offer a balance between performance and image quality, effectively utilizing FP16 computations where they won’t impact the outcome.
Additional Software Optimizations
- VRAM Efficiency: Tools and modifications that improve VRAM efficiency can allow for running Stable Diffusion on systems with less VRAM without a substantial loss in performance or quality.
- Parallel Processing: Utilizing the capabilities of multi-core CPUs or multiple GPUs through parallel processing commands can significantly reduce image generation times.
Hardware and Environmental Adjustments
- Effective Cooling: Maintaining an efficient cooling system ensures that your hardware operates within optimal temperature ranges, preventing thermal throttling and maintaining consistent performance.
- Regular Updates: Keeping all software components, including operating systems, GPU drivers, and the Stable Diffusion application itself, updated can help in leveraging the latest performance improvements and bug fixes.
Incorporating Community Insights
The Stable Diffusion community, particularly on platforms like GitHub and Discord, is a valuable resource for discovering new optimizations. Contributors often share their findings, including command-line tweaks and hardware setups that have yielded improvements in their experience. Engaging with these communities can provide access to cutting-edge tips and optimizations beyond the official documentation.
By applying these command-line arguments and optimizations, users can tailor the performance of Stable Diffusion to better match their system’s capabilities and their individual needs. Whether you’re working with a high-end setup aiming for maximum efficiency or trying to make the most out of a lower-end system, these adjustments can significantly impact your workflow and output quality.
Source: https://github.com/AUTOMATIC1111/stable-diffusion-webui/wiki/Optimizations
Conducting a cost vs. performance analysis for different system specifications can help you make informed decisions when setting up or upgrading your system for Stable Diffusion. This analysis considers the trade-offs between the financial investment in hardware and the expected performance improvement, helping you find the sweet spot for your needs and budget.
Budget Setup
Cost: Low
Performance: Basic functionality with longer processing times and lower output resolutions. Suitable for entry-level experimentation with Stable Diffusion.
- CPU: Quad-core processor (e.g., Intel Core i5 or AMD Ryzen 3)
- GPU: NVIDIA GTX 1050 Ti or equivalent with at least 4GB VRAM
- RAM: 8GB
- Storage: 256GB SSD
Analysis: Budget setups are the most cost-effective option, minimizing initial investment while still allowing access to Stable Diffusion’s capabilities. The trade-off includes longer generation times and limitations on output quality and resolution. This setup is recommended for casual users or those just starting with AI image generation.
Mid-Range Setup
Cost: Moderate
Performance: Balanced, offering faster image generation and higher resolutions. Good for enthusiasts and semi-professional use.
- CPU: 6-core processor (e.g., Intel Core i7 or AMD Ryzen 5)
- GPU: NVIDIA RTX 2060 or AMD RX 5600 XT with 6GB VRAM
- RAM: 16GB
- Storage: 512GB SSD
Analysis: Mid-range setups offer the best balance between cost and performance for most users. They significantly reduce image generation times and allow for higher resolution outputs compared to budget setups. This range is suitable for users who need more power for their projects but are still mindful of their budget.
High-End Setup
Cost: High
Performance: Professional-grade performance with the shortest processing times and the ability to generate very high-resolution images.
- CPU: 8-core processor or higher (e.g., Intel Core i9 or AMD Ryzen 7/9)
- GPU: NVIDIA RTX 3080 or AMD RX 6800 XT with 10GB VRAM or more
- RAM: 32GB or more
- Storage: 1TB NVMe SSD
Analysis: High-end setups require a significant financial investment but offer the best performance for Stable Diffusion. They are capable of handling intensive tasks, multiple instances, and generating high-quality images at speed. Recommended for professionals and power users who require the highest efficiency and output quality.
Recommendations
- For Budget-Conscious Users: Start with a budget setup and consider selective upgrades, such as adding more RAM or opting for a mid-range GPU, to improve performance without a large upfront cost.
- For Enthusiasts and Semi-Professionals: Invest in a mid-range setup. It provides a good balance of performance and cost, suitable for a wide range of tasks without breaking the bank.
- For Professionals and Heavy Users: A high-end setup is advisable. The initial higher cost is offset by significant gains in efficiency, quality, and the ability to handle complex projects with ease.
The choice between budget, mid-range, and high-end setups should be guided by your specific needs, the intensity of your projects, and your budget constraints. Upgrading individual components over time can also be a cost-effective way to gradually improve your system’s performance. Ultimately, investing in the right setup will enhance your experience with Stable Diffusion, enabling you to create more and wait less.
Upgrading your system for Stable Diffusion involves identifying bottlenecks in your current setup and making targeted improvements to enhance performance. The key components to consider upgrading include the GPU, RAM, CPU, and storage. Here’s a strategic approach to upgrading your system for better performance with Stable Diffusion:
1. Upgrade the GPU
- Why It’s Important: The GPU is the most critical component for Stable Diffusion. A powerful GPU accelerates image generation, supports higher resolutions, and improves overall performance.
- What to Consider: Look for GPUs with higher VRAM, as Stable Diffusion can be VRAM-intensive. NVIDIA GPUs are generally recommended due to their CUDA support, which is optimized for deep learning tasks. Upgrading from a lower-end GPU to a mid-range or high-end GPU, such as the NVIDIA RTX 3060, 3070, or even 3080, can offer significant performance improvements.
2. Increase RAM
- Why It’s Important: More RAM allows your system to handle larger datasets and multitask more efficiently without slowing down.
- What to Consider: If you have 8GB, upgrading to 16GB or 32GB can significantly improve performance, especially if you’re running multiple applications or working with large image resolutions in Stable Diffusion.
3. Upgrade the CPU
- Why It’s Important: While the GPU handles most of the heavy lifting for Stable Diffusion, the CPU is essential for overall system performance, affecting everything from the model loading times to preprocessing steps.
- What to Consider: Upgrading to a faster CPU with more cores can reduce bottlenecks, particularly if you’re using a CPU-bound application or running tasks in parallel. Look for modern CPUs with higher clock speeds and additional cores, such as AMD Ryzen 5/7/9 or Intel i7/i9 series.
4. Switch to SSD Storage
- Why It’s Important: An SSD can drastically reduce load times compared to a traditional HDD, speeding up boot times, data transfer, and application launch times, including the loading of Stable Diffusion models.
- What to Consider: Upgrading to a larger and faster SSD not only provides quicker access to data but also offers improved durability and reliability. Consider NVMe SSDs for the best performance.
Additional Tips
- Check Compatibility: Before purchasing any upgrades, ensure they are compatible with your existing system, including motherboard slots for GPUs and RAM, power supply capacity for the GPU, and CPU socket compatibility.
- Cooling System: Upgrading your cooling system (adding more or better fans, considering liquid cooling) can improve performance by preventing thermal throttling, especially important for high-end GPUs and CPUs.
- Software Optimization: Alongside hardware upgrades, optimizing your software settings, updating drivers, and maintaining a clean operating system can also enhance performance.
Strategically upgrading your system can dramatically improve your experience with Stable Diffusion, reducing generation times and enabling higher quality outputs. Start with the component that is most limiting your current performance, typically the GPU or RAM, and consider your specific needs and budget constraints to guide your upgrades. By focusing on these key components, you can ensure a more efficient and enjoyable creative process with Stable Diffusion.
When working with Stable Diffusion, users may encounter various setup and performance issues. Identifying and resolving these issues efficiently can significantly enhance your experience. Below, we provide solutions to some common problems and point you toward resources for further help and community support.
Common Issues and Solutions
1. Insufficient VRAM
- Symptom: Errors related to running out of memory when trying to generate images.
- Solution: Lower the image resolution or batch size. Consider using model optimizations such as FP16 precision or model pruning. If the issue persists, upgrading to a GPU with more VRAM may be necessary.
2. Slow Image Generation
- Symptom: Longer than expected wait times for image generation.
- Solution: Ensure you are using the GPU for processing (not accidentally running on CPU). Update to the latest drivers and CUDA version. Consider closing other applications to free up resources. For NVIDIA users, enabling CUDA and cuDNN can offer significant speed improvements.
3. Installation Errors
- Symptom: Errors during the installation of Stable Diffusion or related software dependencies.
- Solution: Double-check Python, PyTorch, and CUDA compatibility. Ensure you have the correct versions installed for your hardware. Follow the installation guides closely, and consider using a virtual environment to manage dependencies.
4. Model Loading Issues
- Symptom: Errors or extremely long loading times when trying to load the Stable Diffusion model.
- Solution: Verify that the model files are correctly downloaded and not corrupted. Ensure your system meets the minimum RAM and VRAM requirements. Upgrading your storage to an SSD can also reduce loading times.
5. Inaccurate Image Outputs
- Symptom: The generated images do not match the input prompts or expected quality.
- Solution: Refine your input prompts for better clarity or specificity. Experiment with different seeds or model parameters. Ensure you’re using the latest version of the model, as updates may include improvements in image quality and prompt interpretation.
Resources for Further Help and Community Support
- GitHub Issues Page: The GitHub repository for Stable Diffusion or its forks often has an issues page where users report bugs and share solutions. It’s a great place to search for similar problems or post your own.
- Discord Servers: Many AI art and Stable Diffusion communities have active Discord servers where users share tips, seek advice, and offer real-time support.
- Reddit: Subreddits related to Stable Diffusion and AI art are valuable resources for advice, troubleshooting tips, and community insights.
- Official Documentation: Always refer to the official documentation for the version of Stable Diffusion you are using. It often includes troubleshooting sections and FAQs.
- Stack Overflow: For programming and technical issues, Stack Overflow can be a useful resource. Use specific tags related to Stable Diffusion, Python, PyTorch, etc.
Encountering issues while setting up or running Stable Diffusion is common, especially given the complex interplay of hardware and software requirements. By systematically addressing common problems and utilizing available resources for help, you can overcome most challenges. Remember, the community around Stable Diffusion is continually growing, making it easier than ever to find support and solutions to enhance your experience.
Choosing the right system requirements for Stable Diffusion is important for unlocking its full potential in generating high-quality AI art. Whether you’re a hobbyist exploring creative boundaries or a professional requiring efficient and high-resolution outputs, understanding the relationship between hardware capabilities and software configurations is key to a seamless experience. The journey from selecting the appropriate CPU and GPU to optimizing RAM and storage space can significantly impact performance, dictating the speed, quality, and complexity of the images you can create.
The exploration doesn’t end with hardware. The ecosystem surrounding Stable Diffusion, characterized by its diverse forks and user interfaces, offers a tailored experience to meet various user preferences and needs. From Automatic1111’s WebUI known for its comprehensive feature set and user-friendly design to the streamlined simplicity of InvokeAI and the performance-focused adaptations of WebUI Forge, each fork presents unique advantages. Furthermore, tools like Fooocus and ComfyUI emphasize ease of use and accessibility, ensuring there’s an entry point for everyone into the world of AI-generated art.
Optimizations play a critical role in enhancing your Stable Diffusion experience. Leveraging command-line arguments, engaging with community-shared tweaks, and applying software-specific adjustments can transform your creative process. These optimizations help navigate the challenges of limited system resources, enabling smoother operations and faster image generation even on less powerful setups.
As you continue learning Stable Diffusion, remember that the community is one of your greatest resources. From troubleshooting common issues to exploring advanced techniques, the shared knowledge and experiences of others can be invaluable. Whether through GitHub discussions, Discord channels, or dedicated forums, engaging with the community can provide insights, inspire new ideas, and offer support through challenges.
In conclusion, the fusion of the right system requirements with thoughtful software choices and community engagement forms the cornerstone of a rewarding Stable Diffusion experience. We encourage you to explore, experiment, and optimize, continuously pushing the boundaries of what is possible with AI-generated art. Your perfect setup might be just one upgrade or tweak away, opening doors to uncharted territories.
Glossary of Terms
Term | Definition |
---|---|
AI (Artificial Intelligence) | A branch of computer science dealing with the simulation of intelligent behavior in computers. |
CUDA (Compute Unified Device Architecture) | A parallel computing platform and API model created by NVIDIA for computing on NVIDIA GPUs. |
CPU (Central Processing Unit) | The primary component of a computer that performs most of the processing. |
cuDNN (CUDA Deep Neural Network library) | A GPU-accelerated library for deep neural networks provided by NVIDIA. |
FP16 (16-bit Floating Point) | A computation precision level using 16 bits to represent floating-point numbers, balancing performance and accuracy. |
FP32 (32-bit Floating Point) | A computation precision level using 32 bits to represent floating-point numbers, providing higher accuracy. |
GPU (Graphics Processing Unit) | A specialized electronic circuit designed to accelerate image and video rendering. |
HDD (Hard Disk Drive) | A data storage device using magnetic storage to store digital information. |
IDE (Integrated Development Environment) | A software application providing comprehensive facilities to computer programmers for software development. |
Latent Diffusion Models | Generative models that learn to generate new data samples from noise, guided by conditioning input. |
NVMe (Non-Volatile Memory Express) | An interface protocol for SSDs to accelerate data transfer between a computer’s storage and its memory. |
PyTorch | An open-source machine learning library for applications such as computer vision and natural language processing. |
RAM (Random Access Memory) | A form of computer memory used to store working data and machine code. |
SSD (Solid State Drive) | A storage device using flash memory to store data, providing faster access than HDDs. |
VRAM (Video RAM) | Specialized memory used by GPUs to store image data for display; it’s faster than regular RAM. |
Links to Resources for Further Reading and Exploration
Resource | Link |
---|---|
Stable Diffusion GitHub Repository | Visit GitHub |
PyTorch Official Website | Visit PyTorch |
NVIDIA CUDA Toolkit | Visit NVIDIA CUDA |
NVIDIA cuDNN | Visit NVIDIA cuDNN |
Python Official Website | Visit Python |
Visual Studio Code | Visit VS Code |
This table format organizes the key terms and resources, making it easier to reference the concepts and tools associated with Stable Diffusion and its ecosystem.
Stable Diffusion Hardware Requirements:
- System Requirements: Your Complete Guide
- Do you need a GPU for Stable Diffusion?
- What GPU is needed for Stable Diffusion?
- How much RAM do you need for Stable Diffusion?
- Is 2gb VRAM enough for Stable Diffusion?
- Is RTX 3060 good for Stable Diffusion?
- How many GPUs do you need to train Stable Diffusion?
- Is 8GB enough for Stable Diffusion? Is 8GB VRAM overkill?
- Do you need an SSD for Stable Diffusion?
- Can Stable Diffusion run on a CPU?
- Should I use CPU or GPU for Stable Diffusion?
Leave a Reply