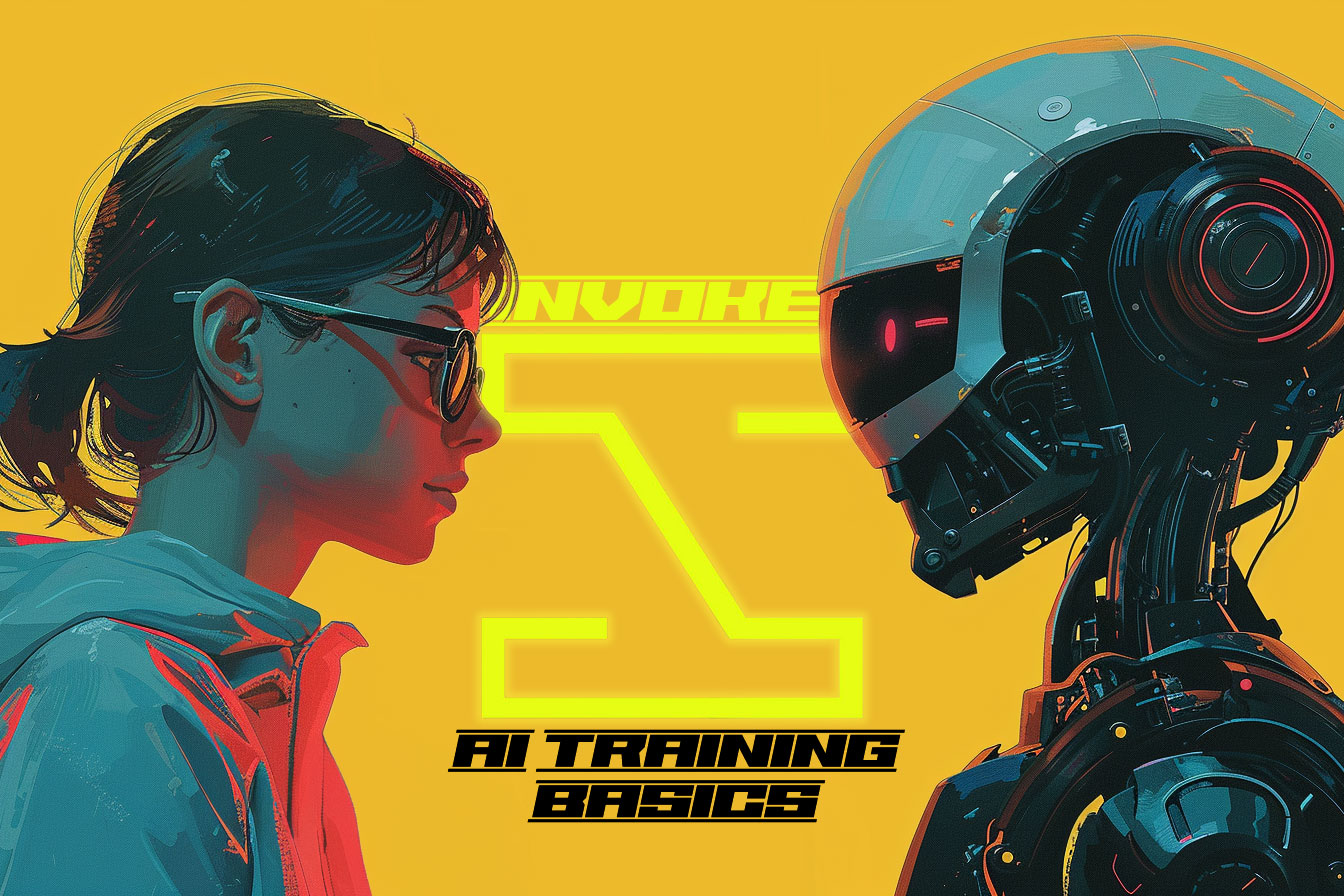
Learn AI Training Basics with Invoke Training for Stable Diffusion
Welcome to Our Detailed Walkthrough: Creating Embeddings and Concept Models with Invoke Training
In this AI training basics guide, we will be focusing on the basic requirements for how to train your own custom AI models in Invoke, a platform well known for its user-friendly interface and methodical integration of functionalities tailored directly to artist’s needs.
Invoke stands out due to its meticulously designed UI and functional approach. Unlike other WebUI’s that push out experimental features, Invoke takes a thoughtful route, releasing additions that are both functional and essential for users working within a production pipeline. This philosophy ensures purposeful enhancements, improving the user experience and addressing specific community needs.
Stable Diffusion AI Training Basics
This guide explores the intricate process of training custom models using Invoke’s open-source scripts. We zero in on two powerful training concepts: embeddings and concept models. Through textual inversion and LoRAs (Low-Rank Adaptation) training, we unlock Invoke’s potential for generating customized and precise outputs.
As we experiment with Invoke’s capabilities, we’ll appreciate its well-designed UI, which fosters an intuitive and efficient workflow. The developer’s commitment to evolving alongside users—without overwhelming unnecessary features—is a commendable approach to user-centric development. By the end of this session, you’ll gain a comprehensive understanding of leveraging Invoke for creating embeddings and concept models, elevating your projects with advanced AI customization.
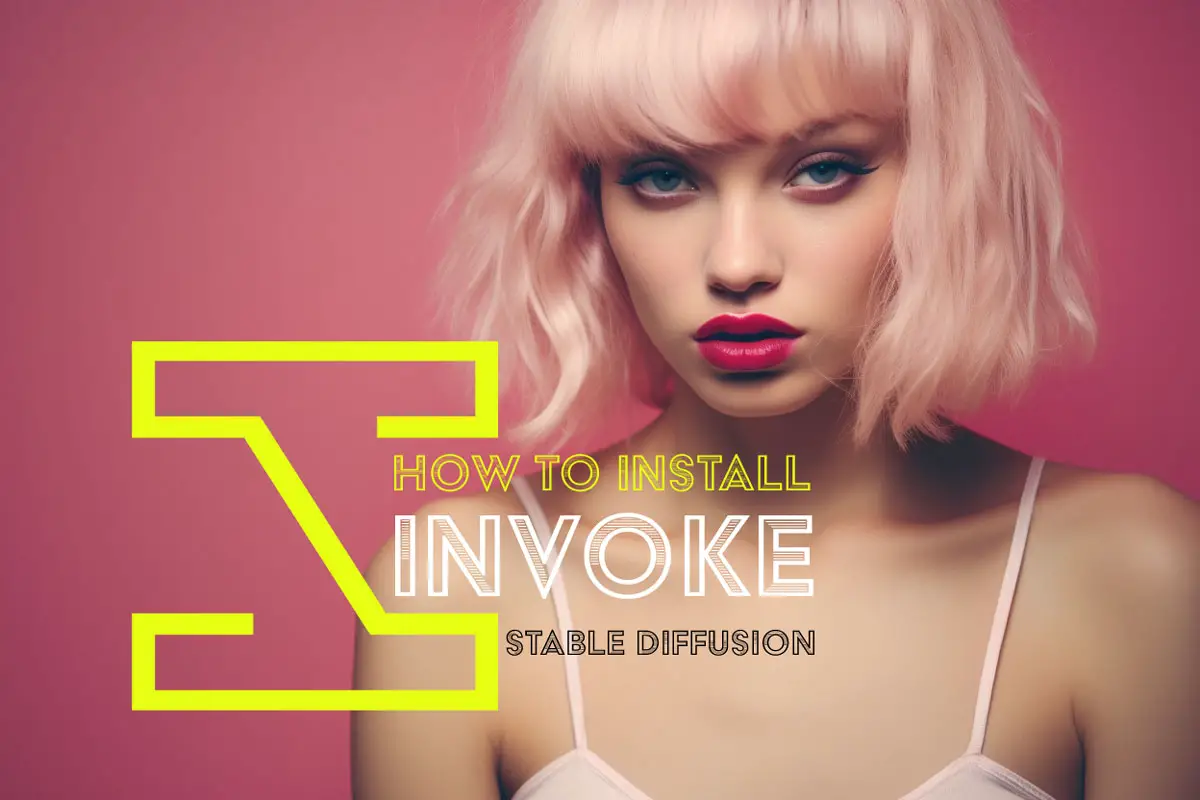
In this tutorial, you will learn how to install Invoke for Stable Diffusion and how to use it to generate amazing images from your own prompts.
Table of Content
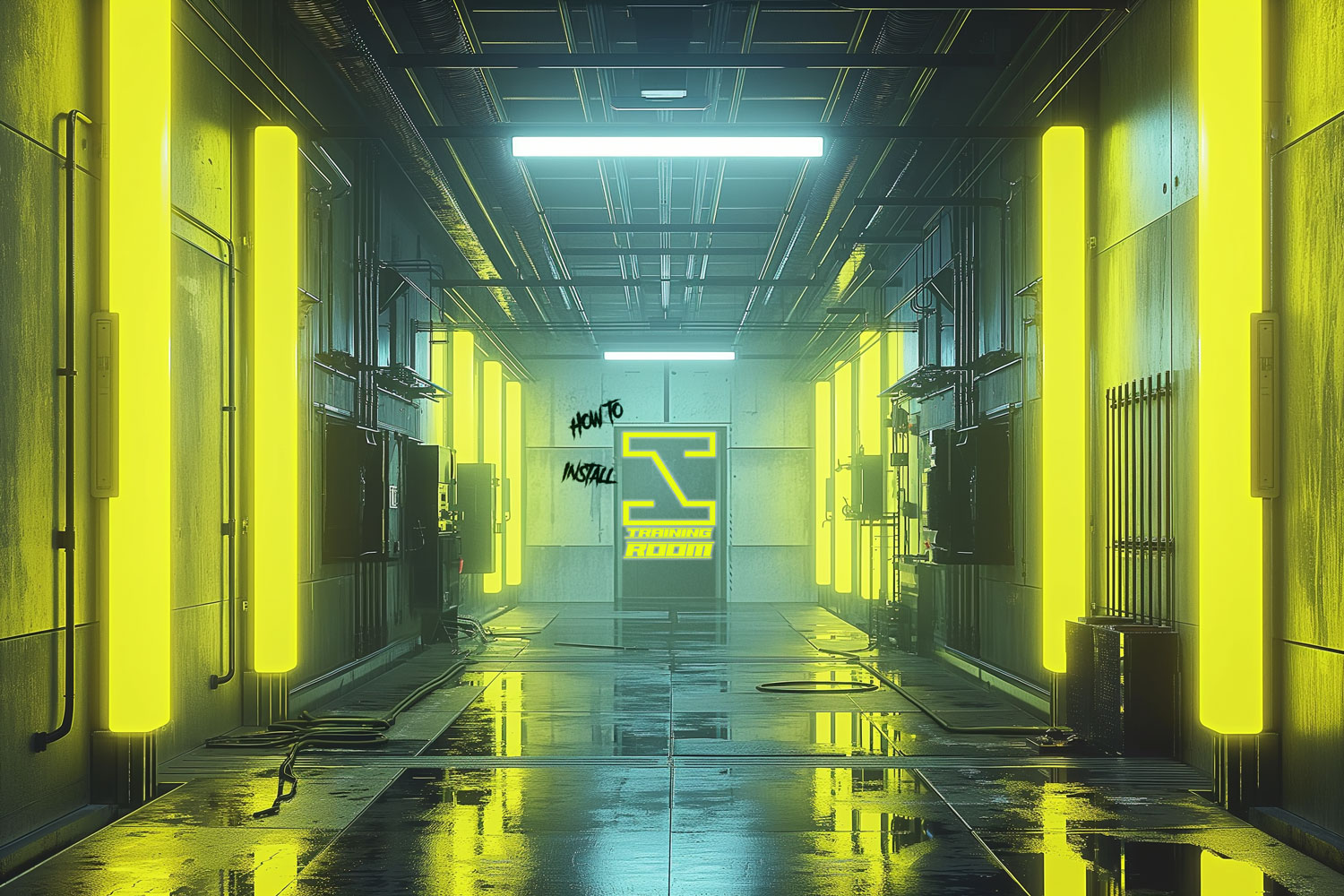
Learn how to install Invoke Training UI with this step-by-step guide. Use this training UI to customize your Stable Diffusion models.
Embeddings and Concept Models
In this section, we shed light on two powerful training methods: embeddings and concept models, providing insights into their functionalities and applications.
Embeddings: The Essence of Personalization
Embeddings serve as a cornerstone in model training, allowing AI models to gain a deeper understanding of specific concepts, themes, or styles. Trained through textual inversion, embeddings customize the model’s perception, enabling it to grasp nuanced details that generic models might overlook.
- Textual Inversion: This process involves training the model to associate unique tokens with specific concepts or attributes. By embedding these associations, textual inversion allows precise manipulation of outputs, tailoring them to specific requirements or creative visions.
- Customization and Precision: Creators can guide the AI’s output more directly using embeddings, ensuring that generated content aligns closely with intended themes or styles. This level of customization opens up new avenues for creative flexibility, resulting in content that feels unique to you.
Embeddings in the context of Stable Diffusion are like giving the AI model a set of secret codes or shortcuts that directly link to specific styles, concepts, or even individual items within its vast knowledge base. Imagine Stable Diffusion as an artist with a sketchbook full of drawings. Each drawing is unique, but explaining how to recreate a specific style or theme from those drawings can be complex. Embeddings simplify this by creating a direct pathway to those specific styles or themes, making it much easier for the model to understand and replicate them upon request.
Here’s a more detailed breakdown:
- Categorize and Encode: If you’ve ever used a hashtag to categorize or find content on social media, you already understand the basic principle of embeddings. In Stable Diffusion, embeddings work similarly but are much more sophisticated. They categorize and encode vast amounts of information about a style, concept, or subject into a compact, easily accessible format. This enables the model to “recall” and use these details efficiently when generating new images.
- Fine-tuning with Textual Inversion: The process of creating embeddings often involves what’s called “textual inversion.” This is where you teach Stable Diffusion new “concepts” or “styles” by training it with a series of images that embody what you’re trying to capture. Through this training, the model learns to associate a new token or series of tokens with these images. Later, when you use these tokens in your prompts, Stable Diffusion understands precisely what you’re asking for and can generate images that match your request more closely.
- Improved Personalization: Embeddings allow you to customize Stable Diffusion’s outputs in incredibly specific ways. Whether you want to generate images in the style of a particular artist, or you’re aiming for a certain aesthetic (e.g., cyberpunk, vaporwave), embeddings give you the power to guide the model’s creativity according to your precise specifications.
- Creative Consistency: One of the significant advantages of using embeddings is achieving a consistent style or theme across multiple image generations. Without embeddings, even small variations in your textual prompts can lead to unpredictably varied outputs. Embeddings ensure that the core attributes you care about are consistently interpreted and represented by the model, leading to more reliable creative results.
In summary, embeddings in Stable Diffusion act as powerful enhancers of the model’s ability to generate images that align closely with your concept. They offer a way to personalize and direct the AI’s generative capabilities, ensuring that the output not only meets but often exceeds your expectations. By utilizing embeddings, you essentially expand the language and understanding between you and Stable Diffusion, fostering a more intuitive and fruitful creative collaboration.
Concept Models: Expanding Your Toolset
Concept models take AI potential a step further by introducing new concepts beyond the model’s original training data. Leveraging DoRA (Weight-Decomposed Low-Rank Adaptation) or LoRA (Low-Rank Adaptation) training, these models redefine the AI’s interpretative framework, enabling it to generate outputs that incorporate novel ideas or elements.
- LoRA Training: Modifications to the model’s attention mechanism allow dynamic reweighting of attention scores. This equips the model to integrate and understand new concepts, effectively expanding its knowledge base.
- Unique Extension: Training concept models pushes the boundaries of what AI can generate, introducing elements previously outside the model’s comprehension. This capability is important for projects requiring unique, unconventional, or innovative ideas.
For those familiar with Stable Diffusion, understanding concept models can be similar to exploring a new expansion pack for your favorite game. Stable Diffusion, as you know, is a powerful text-to-image model that generates images based on textual descriptions. However, its knowledge and creativity are bounded by the data it was trained on. This is where concept models come into play, acting as a targeted update to the model’s capabilities.
Think of a concept model as a specialized plugin or module for Stable Diffusion that introduces it to new concepts or themes it wasn’t originally familiar with. For example, if Stable Diffusion has been trained on a vast array of general imagery and concepts but you want it to generate art in a very specific, perhaps novel style, or imagine new types of futuristic technology, a concept model is your go-to solution.
Here’s a closer breakdown:
- Expanding the Lexicon: Stable Diffusion operates within the confines of its training data. Concept models serve as a bridge to new territories—teaching it about things it hasn’t seen before. It’s like adding new words to its vocabulary, where each word is a concept or idea you want it to understand and visualize.
- Training with Precision: By training a concept model, you’re effectively fine-tuning Stable Diffusion on a subset of data that exemplifies the new concept. This process is akin to giving it a crash course or intensive workshop on a subject, like introducing it to cyberpunk aesthetics or the intricacies of baroque architecture, which were perhaps underrepresented in its original training data.
- Creative Expansion: Once equipped with this new knowledge, Stable Diffusion can not only recognize and reproduce these newly learned concepts but also blend them with its existing knowledge to create something truly unique. For instance, after learning about cyberpunk aesthetics through a concept model, Stable Diffusion can start generating images that mix these aesthetics with other styles or themes it knows, creating art that pushes the boundaries of its original capabilities.
In essence, concept models are a powerful tool for users who want to push Stable Diffusion beyond its baseline creativity, enabling it to generate images that are tailored to very specific or novel ideas. It’s a way of customizing and extending the model’s understanding to align with your creative vision, ensuring that the output is as close as possible to what you imagine.
Combining Embeddings and Concept Models
Combining embeddings and concept models in the context of Stable Diffusion is like assembling a supercharged creative toolkit, where each tool complements and enhances the other, enabling you to push the boundaries of AI-generated art. This powerful combination allows you to both deeply understand specific themes or styles (via embeddings) and expand the AI’s creative capabilities to include new, previously unlearned concepts (via concept models). Let’s dig deeper into how these components work together to elevate your creative projects.
Synergistic Control
- Custom Precision Meets Broadened Horizons: Embeddings give you the precision to dial in on specific styles, textures, or atmospheres, effectively telling Stable Diffusion, “This is exactly what I mean by this term.” Concept models, on the other hand, broaden the AI’s understanding and capability by introducing it to entirely new concepts it wasn’t initially trained on. When you combine the two, you’re equipped to not only specify your creative desires with pinpoint accuracy but also ensure those specifications can include novel ideas and themes.
- Enhanced Versatility and Detail: Think of embeddings as teaching Stable Diffusion a new language of art, style, and expression, while concept models are about expanding the topics it can converse about. Together, they empower the model to generate images that are not only highly detailed and in tune with your specified style but also enriched with concepts and elements it wouldn’t have been able to produce otherwise.
Practical Application
- Creating Unique Artistic Styles: If you’re aiming to create images in a unique style that combines, say, the intricate patterns of Art Nouveau with the futuristic vibes of cyberpunk, embeddings can help encode the detailed stylistic elements of each. A concept model can then be trained to understand how these styles might merge or coexist in imagery that Stable Diffusion has never seen before, facilitating the creation of genuinely novel art.
- Innovative Content Generation: For projects that require generating content beyond current trends or existing genres—like imagining creatures from an undiscovered planet—embeddings can capture specific textures or anatomical features, while concept models can introduce the model to the broader idea of these alien life forms. This combination allows Stable Diffusion to generate highly detailed and consistent images that are both imaginative and coherent with your project’s theme.
Achieving Coherence and Consistency
- Maintaining Creative Direction: By using embeddings and concept models in tandem, you maintain greater control over the AI’s output, ensuring that your creative direction is clearly communicated and adhered to. This is particularly useful for series or collections of images where thematic and stylistic coherence is key.
- Iterative Exploration and Refinement: This combination also supports an iterative creative process. You can start with broad concepts using concept models and then refine the output with embeddings to adjust styles or details, allowing for an exploration of creative ideas that is both wide-ranging and finely tuned.
In summary, combining embeddings with concept models in Stable Diffusion represents a holistic approach to leveraging AI for creative expression. It combines the depth and specificity of embeddings with the expansive, horizon-broadening nature of concept models, enabling creators to explore new realms of possibility while maintaining a high degree of control and precision over the generated content. This synergy not only enhances the quality and relevance of the AI’s outputs but also opens up new pathways for creative innovation and exploration.
Understanding Training Control
In this important section, we will learn about the foundational aspects of AI model training: Prompt & Text Encoding and Model Weights & Interpretation. These components are vital for anyone looking to grasp the mechanics of training custom models and leverage Invoke’s capabilities to their fullest. Understanding how your prompt interacts with the model’s weights, and subsequently how this affects the generation process, is important for grasping AI-generative art. Let’s explore the nuances of this relationship.
Prompt & Text Encoding
The training process begins with a critical step known as tokenization and text encoding. This process involves breaking down the prompts—inputs provided to the model—into smaller, analyzable pieces. Here’s what makes it essential:
- Tokenization: It transforms the input prompt into tokens, which are smaller parts that the AI model can easily process. This step is pivotal because it converts human-readable text into a format that machines can understand and analyze.
- Text Encoding: Following tokenization, each token is encoded into numerical values. This encoding is crucial as it translates tokens into a mathematical language, enabling the AI model to perform computations and generate predictions based on the input.
The intricacies of tokenization and text encoding are what allow the model to interpret and manipulate the prompts effectively, laying the groundwork for personalized model training. This specific process is something that may change over time, but at it stands right now, this is how you will be managing and manipulating prompt information to pass into models.
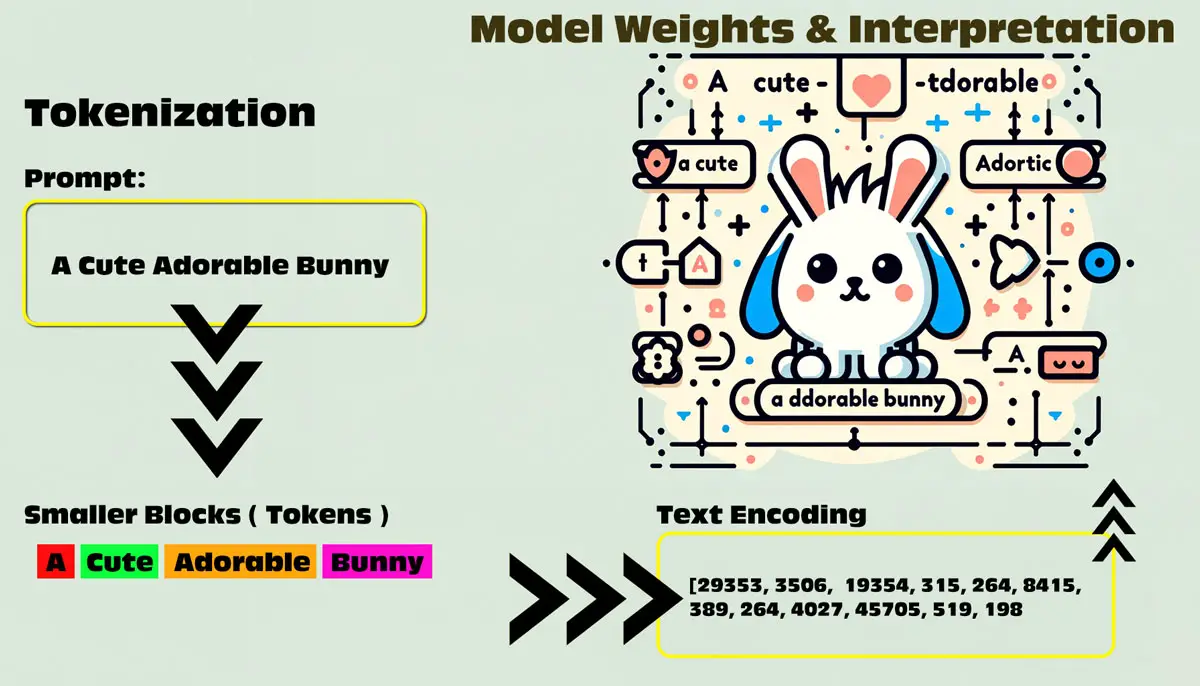
Tokenization:
- Tokenization is the process of breaking down text into smaller units called tokens. These tokens can be words, phrases, or even individual characters. It’s an essential step in the processing of text by language models.
- In the case of Stable Diffusion, tokenization is done using the CLIP model (Contrastive Language-Image Pre-Training). CLIP converts images into detailed descriptions, and its built-in tokenizer is used for this task.
- When you provide a text prompt to Stable Diffusion, it tokenizes that prompt into a sequence of tokens. For example, if your prompt is “a cute adorable bunny,” it would split it into tokens like “a,” “cute,” “adorable,” and “bunny”.
- These tokens serve as the input for Stable Diffusion, allowing it to analyze and generate text based on the context and relationships between individual tokens. So, tokenization helps describe your words or ideas more clearly and enables Stable Diffusion to produce an output closer to your prompt.
Tokenization is like breaking down a complex sentence into smaller building blocks, making it easier for the model to understand and generate meaningful content. Each fine-tuned model are trained to understand prompts and text encoding differently, that is why not all models output the same visual content.
Model Weights & Interpretation
After the initial encoding, the focus shifts to the model weights and how they interpret the encoded text. This stage is where the magic happens:
- Model Weights: Think of these as the brain of the AI model. They are parameters that determine how the model interprets the relationship between different tokens. The weights are adjusted during the training process, refining the model’s ability to generate accurate and relevant outputs.
- Interpretation: The interpretation of prompts by the model is heavily influenced by its weights. It’s a dynamic process where the encoded text (transformed prompts) interacts with the model weights, guiding the generation of outputs. This interaction is akin to light passing through a lens, where the shape of the lens (model weights) affects the resulting image (output).
Understanding these components is akin to learning the language of AI model training. It allows creators to fine-tune their inputs and better guide the training process, achieving results that are more aligned with their creative vision.
By mastering Prompt & Text Encoding and Model Weights & Interpretation, users of Invoke gain deeper insights into the mechanics of AI, enabling them to craft more precise and effective models. This knowledge not only enhances the user’s ability to utilize Invoke’s features but also paves the way for innovation and creativity in their projects.
Dataset Management
Dataset Management is a cornerstone of training and refining AI models like Stable Diffusion, acting as the bedrock upon which the AI’s learning and creative capabilities are built. This process involves not just the collection and organization of data but also its preparation and optimization for training purposes. In the context of AI art generation, managing your dataset effectively can significantly influence the quality, diversity, and accuracy of the outputs. Let’s explore this process in great detail.
Dataset Collection
- Diverse Sources: Begin by gathering a wide range of images or text that aligns with your project’s goals. For art generation, this could mean collecting artworks across various styles, periods, or themes. The key is diversity; the more varied your dataset, the more versatile the AI model becomes.
- Quality and Resolution: Focus on the quality and resolution of the images. High-resolution images ensure that the model learns from the best possible examples, capturing nuances in style and detail that lower-resolution images might miss.
- Legal and Ethical Considerations: It’s crucial to consider the legal and ethical implications of your dataset. Ensure you have the rights to use the images for training purposes and consider the impact of your dataset’s composition on the model’s outputs, avoiding biases or unethical content.
Dataset Organization
- Structured Directories: Organize your dataset into structured directories or folders, possibly categorizing images by style, artist, or content. This organization will simplify the process of training different models or testing the model’s performance across various art styles.
- Metadata and Annotation: Alongside the images, maintain a metadata file that contains information about each image (e.g., artist, style, period). If working with text, annotations that describe the images or text passages can be invaluable for training models to understand context or generate descriptive content.
- Data Cleaning: This step involves removing duplicates, corrupted files, or irrelevant images from your dataset. Cleaning ensures the efficiency and effectiveness of the training process, preventing the model from learning from poor-quality or irrelevant examples.
Data Preparation
- Preprocessing: Before training, images might need preprocessing to fit the model’s input requirements. This could involve resizing images, converting them into a specific format, or normalizing the data to ensure consistency across the dataset.
- Augmentation: Data augmentation involves creating variations of your dataset images through rotation, scaling, or color adjustment. This process can help increase the diversity of your dataset without needing to collect more data, enhancing the model’s robustness and ability to generalize from the training data.
- Partitioning: Split your dataset into training, validation, and test sets. This partitioning is critical for evaluating the model’s performance and preventing overfitting. The training set is used for the AI to learn from, the validation set to tune the model parameters, and the test set to evaluate its final performance.
Dataset Utilization and Refinement
- Training and Feedback Loop: Use the organized and prepared dataset to train your AI model. Monitor the training process closely, using feedback from the validation set to adjust the model’s parameters or the training strategy as needed.
- Iterative Refinement: Training an AI model, especially for creative tasks, is often an iterative process. Based on the outputs and performance metrics, you may need to go back and adjust your dataset—perhaps by adding more examples of a particular style or removing images that lead to undesirable outputs.
- Expansion and Update: As your project evolves, so too should your dataset. Continually adding new data, based on emerging trends, new artistic styles, or feedback from the model’s outputs, will help keep the model’s capabilities fresh and aligned with your creative goals.
In summary, effective Dataset Management is a multifaceted and ongoing process that plays an important role in the success of AI-driven art generation projects. By meticulously collecting, organizing, preparing, and refining your dataset, you provide a solid foundation for training AI models that are capable of producing high-quality, diverse, and creatively fulfilling outputs.
Embeddings: Creating Precise References
Embeddings are essentially about creating a highly focused dataset that serves as a reference point for specific subjects or styles. This process is similar to teaching the AI model a new language, with each term in this language (an embedding) representing a complex set of ideas, styles, or visual elements.
- Dataset for Embeddings: When preparing a dataset for embeddings, you’re gathering images that exemplify the particular subject or style you wish to encode. This dataset doesn’t need to be vast but must be very representative of the concept you aim to capture. For instance, if you’re focusing on “watercolor” as a style, your dataset should include a variety of images that showcase the breadth and diversity of watercolor art.
- Training for Specificity: The goal is to train the AI model to associate a new token—essentially a shortcut or codeword—with the rich, detailed information contained in your dataset. Once trained, invoking this token in prompts allows the model to generate images that exhibit the qualities of your dataset, be it the fluidity of watercolor paintings or the specific anatomy of an animal.
Concept Models: Broadening Stable Diffusion’s Creative Capabilities
While embeddings focus on precision, concept models aim to broaden the AI’s understanding, enabling it to interpret and generate content based on new or expanded concepts.
- Dataset for Concept Models: Creating a concept model requires not just images but also captions that explicitly describe what each image represents. This dual input helps redefine the model’s foundational understanding of prompts. If you’re introducing a “NeoWatercolor” style, your dataset needs to include images showcasing this style across various subjects, alongside captions that highlight key characteristics.
- Captions as Redefinitions: Each caption should serve as a mini-lesson on the new style or subject, guiding the AI to recognize and reproduce the “NeoWatercolor” style when it encounters related prompts. The specificity and clarity of your captions directly influence how well the model assimilates the new concept.
- Variation and Volume: A concept model thrives on variation. The more examples it has, showcasing the concept in different contexts, the more robustly it can understand and generate content related to that concept. While a smaller dataset might suffice for textual inversion and embeddings, concept models benefit from larger datasets—ranging from 20-40 images at the minimum to 100-200 for more comprehensive learning.
Integrating Embeddings & Concept Models into Dataset Management
Managing datasets for embeddings and concept models involves a nuanced approach to selection, organization, and annotation. It’s not just about the quantity of data but its quality and representativeness. Here’s how to integrate these into your Dataset Management strategy:
- Selective Collection: Focus on collecting images that not only represent the concept or style accurately but also bring out subtle nuances that could inform the model’s understanding.
- Structured Annotation: For concept models, the annotations or captions you provide are as crucial as the images themselves. They should be clear, descriptive, and varied, offering insights into the concept or style you’re teaching the model.
- Iterative Refinement: Both embeddings and concept models may require you to revisit and refine your datasets based on the outcomes. This iterative process ensures that your AI model continues to evolve in its understanding and capability.
In sum, managing datasets for embeddings and concept models is a delicate balance of precision, breadth, and depth. By meticulously curating and annotating your datasets, you empower your AI model to generate art that transcends its original programming, offering truly innovative and personalized creations.
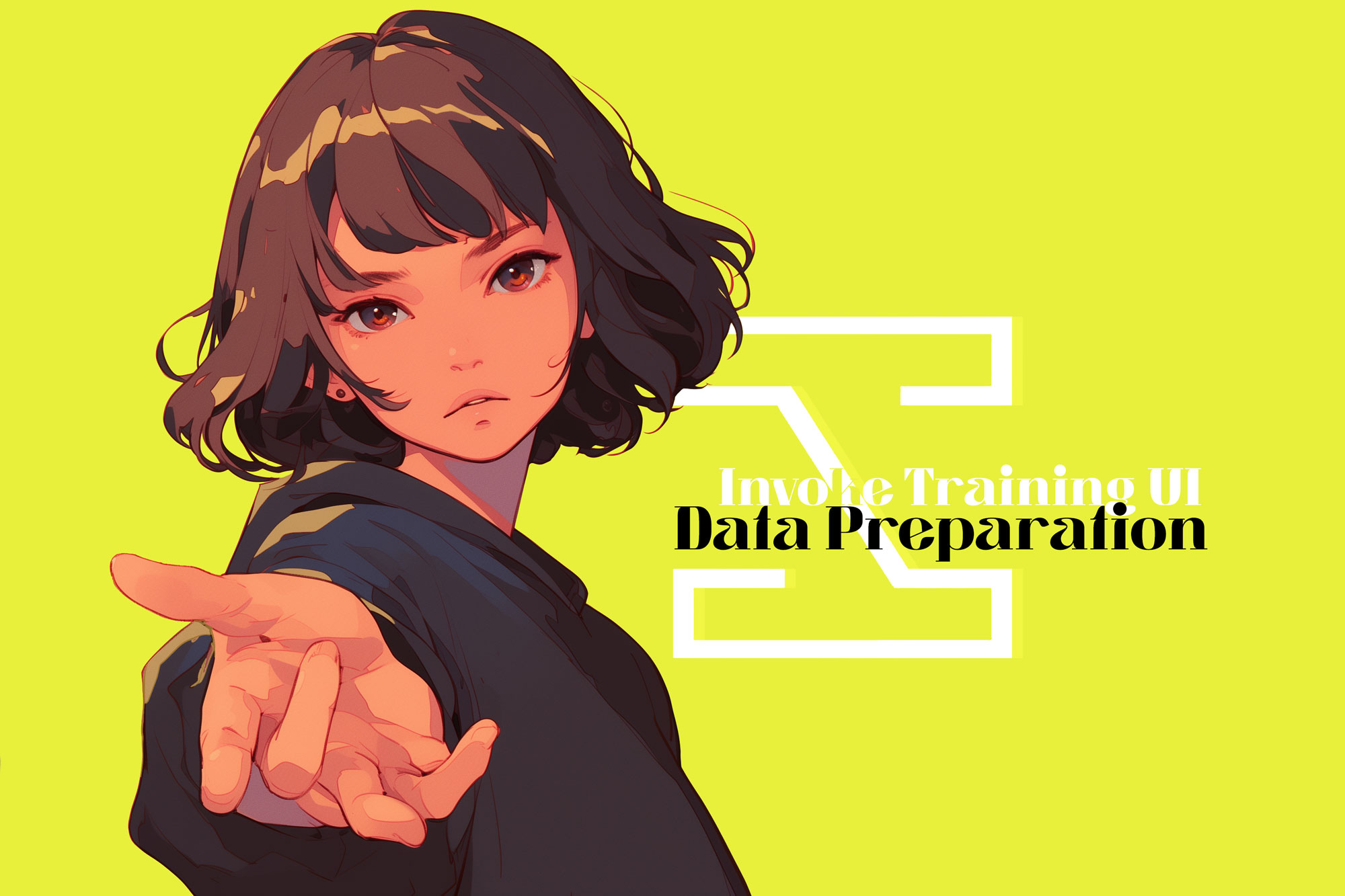
In this guide, we will be preparing datasets with Invoke Training UI. Learn how to effectively create data annotations and datasets for Stable Diffusion.
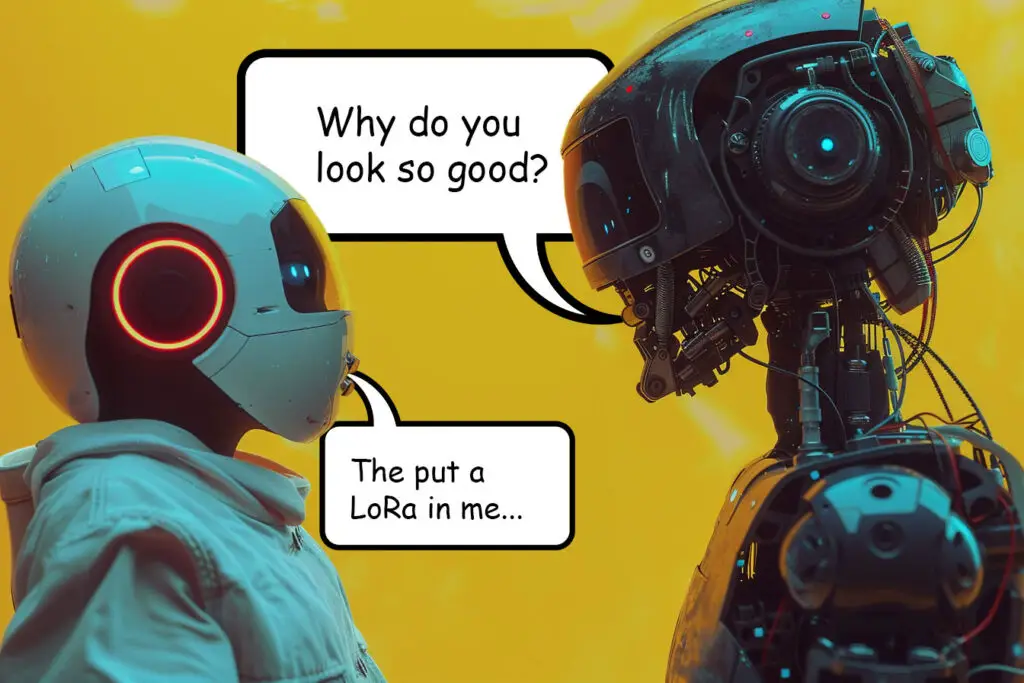
Invoke Training UI-Datasets
What’s the Difference Between LoRa and Embeddings?
- LoRA (Low-Rank Adaptation):
- Definition: LoRA models are smaller versions of Stable Diffusion models that apply smaller modifications to standard checkpoint models.
- Purpose: They allow you to impart a particular style to an image or create a specific character in the generated picture.
- File Size: LoRA models have significantly reduced file sizes, ranging from 2 to 500 MBs, compared to the larger checkpoint files.
- Usage: You can insert LoRA models in real-time into other models, making them portable across different contexts.
- Example: If you want to add a specific artistic style or tweak certain features in an image, LoRA is a powerful tool.
- Embeddings (Textual Inversion):
- Definition: Embeddings modify the text representation (embedding) used by the model. They optimize the embedding to match specific concepts in the image.
- Purpose: They enhance image quality by using negative prompts or fine-tuning specific aspects.
- File Size: Embeddings are tiny files, stored in an extremely small size (e.g., 50-100 KB).
- Usage: You can use embeddings to improve image quality, create specific visual effects, or even generate images related to a particular concept.
- Example: Suppose you want to make a face resemble a specific actor or evoke a certain season—embeddings can achieve this.
In summary, LoRA provides more original data and is adaptable across different models, while embeddings are efficient for specific modifications and quality enhancements. Feel free to test me further—I’m up for the challenge!
Scenarios where you might choose LoRA over embeddings, and vice versa:
- Using LoRA (Low-Rank Adaptation):
- When to Choose LoRA:
- Artistic Style Transfer: If you want to apply a specific artistic style to an image, LoRA is a great choice. For instance, if you’re transforming a photograph into a painting in the style of Van Gogh or Picasso, LoRA can help achieve that unique look.
- Fine-Tuning Specific Features: When you need to tweak certain features in an image, LoRA allows you to make targeted adjustments. For example, enhancing the texture of fur in an animal photo or emphasizing specific architectural details.
- Portable Modifications: LoRA models are smaller and more portable. You can insert them into other models in real-time, making them adaptable across different contexts.
- Example Scenarios:
- Creating Artistic Variations: Suppose you’re building an app that generates personalized art based on user photos. LoRA can add diverse artistic styles to those images.
- Customizing Filters: In a photo editing application, LoRA can provide unique filters that go beyond standard presets.
- When to Choose LoRA:
- Using Embeddings (Textual Inversion):
- When to Choose Embeddings:
- Quality Enhancement: If you’re aiming to improve image quality, embeddings are effective. They optimize the text representation (embedding) used by the model, resulting in better visual output.
- Specific Concept Generation: When you want to generate images related to a particular concept, embeddings shine. For instance, creating visuals associated with specific keywords like “sunset,” “vintage,” or “nostalgia.”
- Efficiency and Compactness: Embeddings are tiny files, making them efficient for specific modifications without bloating the model.
- Example Scenarios:
- Image Captioning: Embeddings can enhance the quality of captions generated for images. They ensure that the textual description aligns well with the visual content.
- Concept-Driven Art: Suppose you’re building an app that generates custom wallpapers based on user preferences. Embeddings can create visuals related to themes like “beach,” “mountains,” or “cityscape.”
- When to Choose Embeddings:
In summary, LoRA provides more originality and adaptability, while embeddings excel in quality enhancement and concept-driven modifications. The choice depends on your specific use case and priorities.
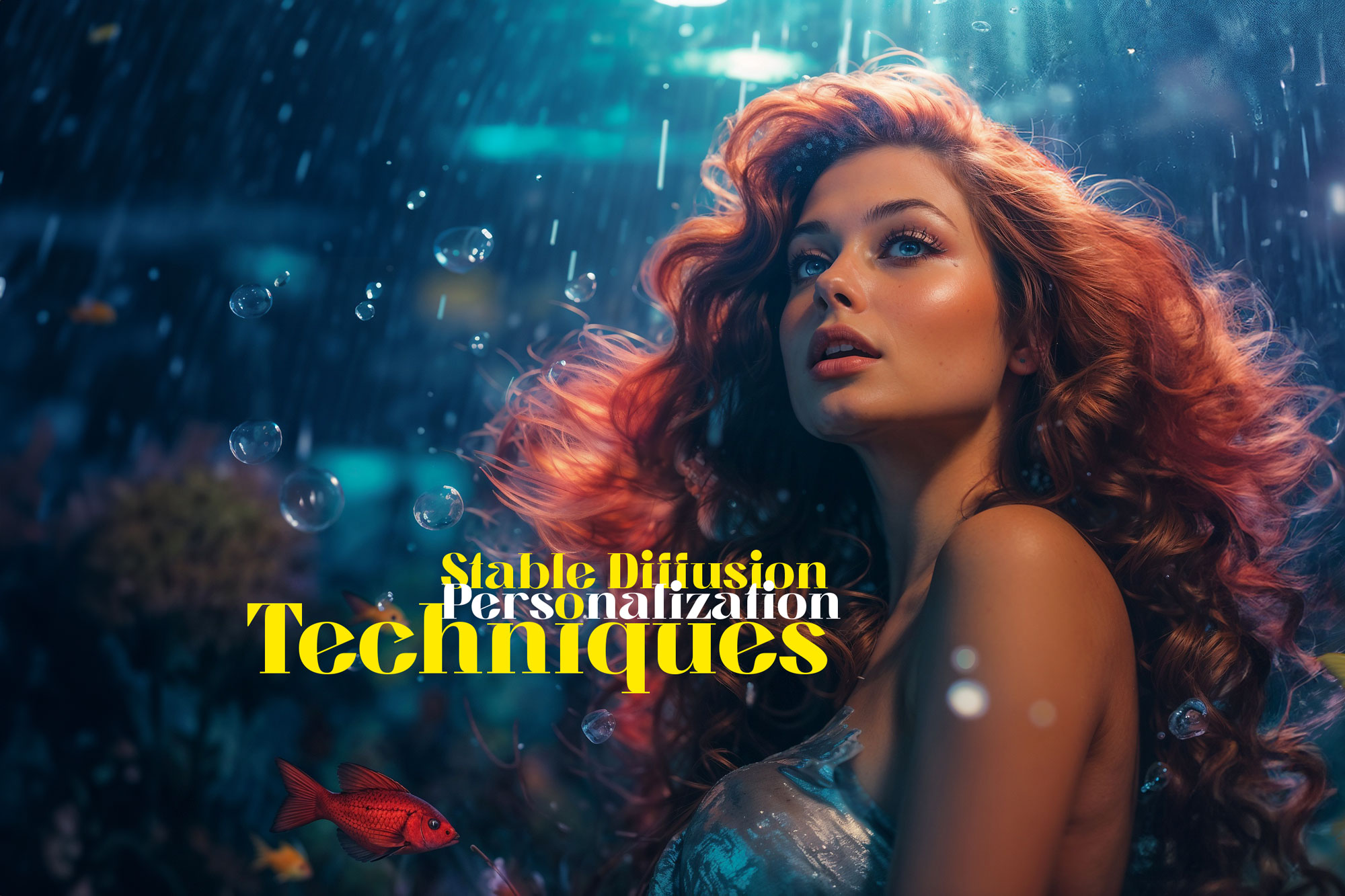
The ability to train custom models has become a vital skill across various fields. As AI continues to integrate into creative and technical domains, data annotation and model training are set to become valuable skill sets and some of the most sought-after professions. Within the creative field, we often use diffusion training, but when it comes to open-source localization, we have several options to choose from: Dreambooth vs LoRA vs Textual Inversion vs Hypernetworks. It can be a bit confusing, so which one should you use?
Next: Invoke Training User Interface
In this guide we learned the AI Training Basics with Invoke Training for Stable Diffusion. In the upcoming guide, we’ll dive into the Invoke Training User Interface and learn about all the settings and parameters to get you started training a textual inversion. You may feel overwhelmed by the assortment of tabs presented, fearing a steep learning curve. However, the reality is much simpler. See you there!
Leave a Reply