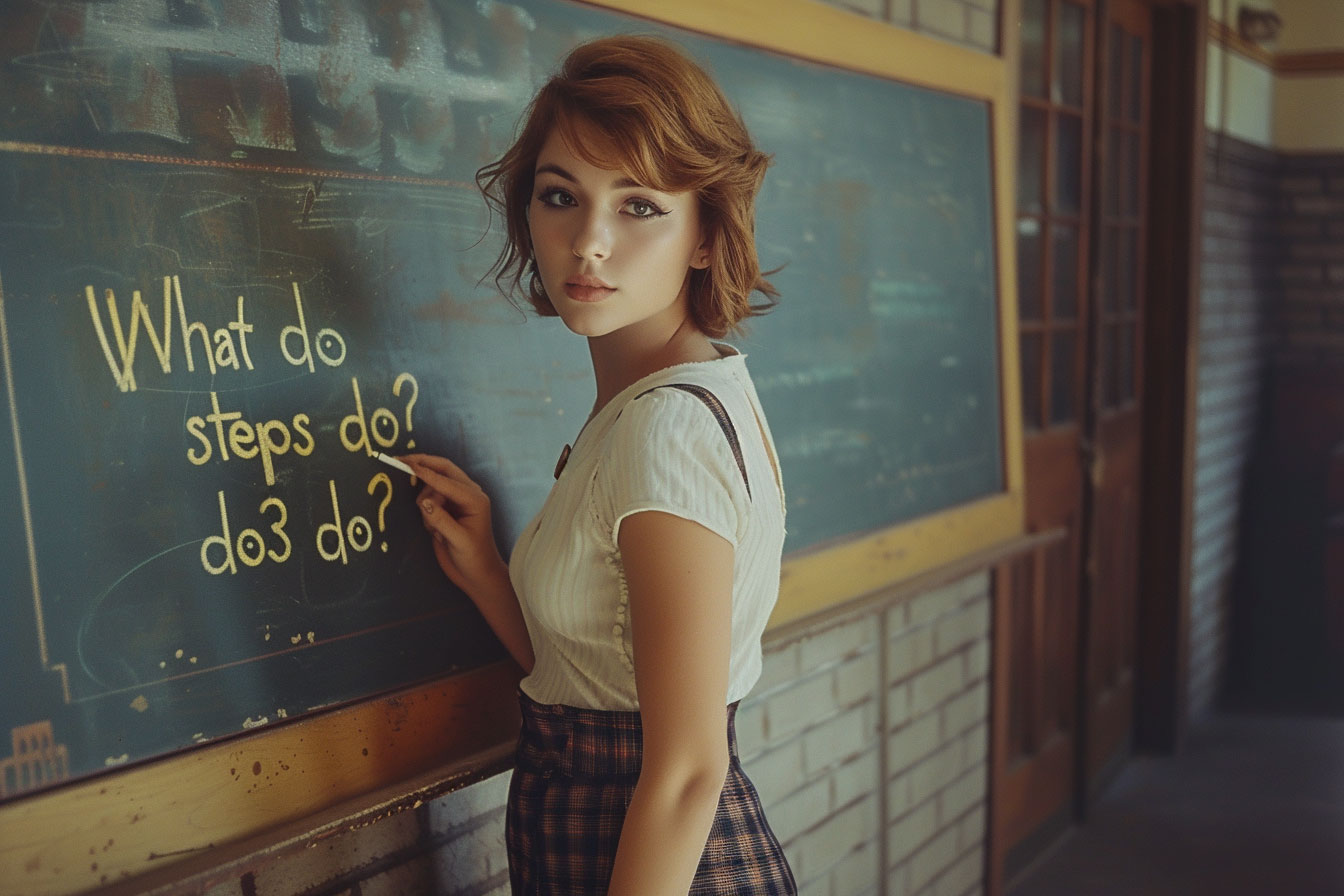
What Do Steps Do in Stable Diffusion? Understand and Learn how to Use Steps
Stable diffusion is a novel technique that uses artificial intelligence to create realistic and diverse images from natural language descriptions. It has many applications in art, design, education, entertainment, and more. But how does it work, and what are the factors that influence the quality and style of the output images? One of the most important factors is the number of steps in Stable Diffusion, but what do steps do in Stable Diffusion?
What Do Steps Do in Stable Diffusion?
What do steps do in Stable Diffusion? Steps are the number of iterations that the AI performs to generate an image from a text prompt using Stable Diffusion. Each step removes some noise from the image and makes it more coherent and detailed. The more steps, the longer it takes to generate the image, but the higher the quality and resolution. However, too many steps can also introduce unwanted artifacts or distortions. The optimal number of steps depends on the model, the sampler, the prompt, and the desired output. According to some users, 20 to 50 steps are usually sufficient for most cases. Source
In this blog post, we will explain what steps are and how they affect the image generation process in stable diffusion. We will also show you some examples of images generated with different numbers of steps and give you some tips and recommendations for finding the optimal number of steps for your goals.
Table of Contents
Stable Diffusion Settings and Parameters
Stable diffusion is a technique that uses a neural network model to generate images from text prompts. The model is trained on a large dataset of images and captions, and learns to associate words with visual features. To generate an image, the model starts with a random noise image and gradually refines it until it matches the text prompt. This process is called diffusion, and it can be controlled by several settings and parameters.
Some of the most common settings and parameters are:
Model:
The model is the neural network that performs the diffusion. There are different models available, such as CLIP, DALL-E, BigGAN, etc. Each model has its own strengths and weaknesses, and can produce different styles and qualities of images. You can choose the model that best suits your needs and preferences.
Sampler:
The sampler is the algorithm that determines how the noise image is updated at each step of the diffusion. There are different samplers available, such as DDIM, U-Net, ResNet, etc. Each sampler has its own advantages and disadvantages, and can affect the speed and stability of the diffusion. You can choose the sampler that best balances the trade-offs between performance and quality.
Temperature:
The temperature is a parameter that controls the randomness and diversity of the diffusion. A higher temperature means more randomness and diversity, but also more noise and distortion. A lower temperature means less randomness and diversity, but also more coherence and fidelity. You can choose the temperature that best matches your desired level of creativity and realism.
Steps:
The steps are the number of iterations that the model performs to generate the image. A higher number of steps means more refinement and detail, but also more time and memory. A lower number of steps means less refinement and detail, but also less time and memory. You can choose the number of steps that best satisfies your expectations and constraints.
What are Steps in Stable Diffusion
Steps are the number of iterations that the model performs to generate the image from the text prompt. Each step removes some noise from the image and makes it more coherent and detailed. The more steps, the longer it takes to generate the image, but the higher the quality and resolution. However, too many steps can also introduce unwanted artifacts or distortions. The optimal number of steps depends on the model, the sampler, the prompt, and the desired output.
How do Steps Work in Stable Diffusion?
To understand how steps work in stable diffusion, we need to understand how diffusion works. Diffusion is a process that starts with a random noise image and gradually refines it until it matches the text prompt. The model learns to reverse the diffusion process by adding noise to the target image and reconstructing it from the noisy image. The model then uses the reconstruction to guide the diffusion process and generate the output image.
The steps are the number of times that the model adds and removes noise from the image. Each step consists of two phases: a forward phase and a backward phase. In the forward phase, the model adds some noise to the image, making it more blurry and noisy. In the backward phase, the model removes some noise from the image, making it more clear and sharp. The model repeats these two phases until it reaches the desired number of steps.
How do Steps Affect the Image Generation Process in Stable Diffusion?
The steps determine how much noise is added and removed from the image at each iteration. A higher number of steps means that the model adds and removes less noise at each step, resulting in a smoother and more gradual diffusion process. A lower number of steps means that the model adds and removes more noise at each step, resulting in a faster and more abrupt diffusion process.
The steps also determine the quality and resolution of the output image. A higher number of steps means that the model has more time and information to refine the image and make it more realistic and detailed. A lower number of steps means that the model has less time and information to refine the image and make it more abstract and low-resolution.
The steps are one of the most important factors that influence the image generation process in Stable Diffusion. They can affect the speed, stability, diversity, and creativity of the output images. In the next section, we will show you some examples of images generated with different numbers of steps and compare them.

Have you ever wondered what it would be like to create images from your imagination, just by typing some words? Imagine being able to generate realistic photos, artistic paintings, or anything in between, in seconds. This is reality and by now most of us are familiar with it. At the rate this technology is growing, it will transform how we work forever. It might just be a change that is as big as the adoption of the internet, thanks to a technology called Stable Diffusion.
Examples of Images Generated with Different Steps
In this section, we will show you some examples of images generated with different numbers of steps and compare them. We will use the same text prompt and the same model and sampler for all the examples, but vary the number of steps. To follow along, I’ve provided you with the prompts and necessary information below.
The text prompt is “a cute cat wearing a hat”. The model is CLIP and the sampler is DDIM. Here are the images generated with 10, 20, 30, 40, and 50 steps, respectively:
Prompt: Photo of a burger with cheese from food photograph, food photography, photorealistic, ultra realistic, maximum detail, foreground focus, recipes.com, epicurious, instagram, 8k, volumetric light, cinematic, octane render, uplight, no blur, depth of field, dof, bokeh |
Negative Prompt: (worst quality, low quality, normal quality, lowres, low details, oversaturated, undersaturated, overexposed, underexposed, grayscale, bw, bad photo, bad photography, bad art)++++, (watermark, signature, text font, username, error, logo, words, letters, digits, autograph, trademark, name)+, (blur, blurry, grainy), morbid, ugly, asymmetrical, mutated malformed, mutilated, poorly lit, bad shadow, draft, cropped, out of frame, cut off, censored, jpeg artifacts, out of focus, glitch, duplicate, (airbrushed, cartoon, anime, semi-realistic, cgi, render, blender, digital art, manga, amateur)++, (3D ,3D Game, 3D Game Scene, 3D Character), (bad hands, bad anatomy, bad body, bad face, bad teeth, bad arms, bad legs, deformities)++ |
Juggernaut Final: Download it here. | Sampling Method: DPM++ 2M Karras |
CFG Scale: 7 | Seed: 1230626881 |
Width: 512 | Height: 512 |


I’ve written a guide on how to use the XYZ plot like the one in the images above. To learn how to create these, check out my guide below.

Successful AI creations hinge not only on talent, but also on the effective use of sophisticated tools. Among them, the XYZ Plot of Stable Diffusion shines bright. This tool streamlines your creative process, offering a tangible roadmap for your AI visual generation. How do you use XYZ plot in Stable Diffusion? The XYZ plot, found…
As you can see, the images become more clear and detailed as the number of steps increases from steps 1-10. Depending on the model you use, it gets to a point where the improvements get diminishing returns or introduce unwanted artifacts.
With the Juggernaut Final model you hardly see improvements in quality beyond 10.
However, you may also notice that there are slight changes and different variations.
This shows that there is a trade-off between quality and diversity when using steps in Stable Diffusion. A higher number of steps means more quality and detail, but also more monotony and conformity. A lower number of steps means more abstraction and noise, but also more creativity and diversity. You have to decide what kind of output you want and choose the appropriate number of steps accordingly. You not notice the difference too much in the burgers above, but below, you will see examples of the the different effects each step has in various samplers and different models.

In the next section, we will discuss some of the challenges and limitations of using steps in stable diffusion.
Challenges and Limitations of Using Steps in Stable Diffusion
In this section, we will discuss some of the challenges and limitations of using steps in stable diffusion. Steps are a powerful and flexible parameter that can influence the quality and diversity of the output images, but they also come with some drawbacks and constraints. Some of the most common challenges and limitations are:
Speed:
The speed of the image generation process depends on the number of steps. A higher number of steps means a longer diffusion process, which can take several minutes or even hours to complete. A lower number of steps means a shorter diffusion process, which can take a few seconds or minutes to complete. The speed also depends on the model, the sampler, the temperature, and the hardware. You have to consider the trade-off between speed and quality when choosing the number of steps.
Memory:
The memory of the image generation process depends on the number of steps. A higher number of steps means a larger memory footprint, which can consume a lot of RAM and disk space. A lower number of steps means a smaller memory footprint, which can save some RAM and disk space. The memory also depends on the model, the sampler, the temperature, and the hardware. You have to consider the trade-off between memory and quality when choosing the number of steps.
Artifacts:
The artifacts of the image generation process depend on the number of steps. A higher number of steps means more refinement and detail, but also more risk of introducing unwanted artifacts or distortions. A lower number of steps means less refinement and detail, but also less risk of introducing unwanted artifacts or distortions. The artifacts also depend on the model, the sampler, the temperature, and the prompt. You have to consider the trade-off between artifacts and quality when choosing the number of steps.
These are some of the challenges and limitations of using steps in stable diffusion. They can affect the performance, efficiency, and reliability of the image generation process. You have to be aware of them and adjust the number of steps accordingly. In the next section, we will give you some tips and recommendations for finding the optimal number of steps.
Tips and Recommendations for Finding the Optimal Number of Steps
Here, we will give you some tips and recommendations for finding the optimal number of steps for your image generation goals. There is no definitive answer to what is the best number of steps, as it depends on many factors, such as the model, the sampler, the temperature, the prompt, the output, and your personal preference. However, here are some general guidelines that can help you choose the right number of steps:
Experiment:
The best way to find the optimal number of steps is to experiment with different numbers and see the results. You can use the preview feature to see how the image changes as the number of steps increases or decreases. You can also compare the images generated with different numbers of steps and evaluate their quality and diversity. You can then choose the number of steps that produces the most satisfying output for you.
Balance:
The optimal number of steps is a balance between quality and diversity, speed and memory, and realism and creativity. You have to consider the trade-offs and constraints of using steps in stable diffusion and find the sweet spot that meets your expectations and needs. You can use the following ranges as a reference:
- 10 to 20 steps: This range is suitable for quick and abstract outputs, with low quality and high diversity. It is good for testing and exploring ideas, but not for final products.
- 20 to 30 steps: This range is suitable for moderate and recognizable outputs, with medium quality and medium diversity. It is good for sketching and prototyping, but not for high-resolution products.
- 30 to 40 steps: This range is suitable for realistic and detailed outputs, with high quality and low diversity. It is good for finalizing and polishing, but not for creative products.
- 40 to 50 steps: This range is suitable for very realistic and very detailed outputs, with very high quality and very low diversity. It is good for professional and realistic products, but not for original products.
Adjust:
The optimal number of steps is not fixed, but can vary depending on the situation and the goal. You can adjust the number of steps according to the model, the sampler, the temperature, the prompt, and the output. For example, you may need more steps for complex or vague prompts, or less steps for simple or specific prompts. You may also need more steps for high-resolution or realistic outputs, or less steps for low-resolution or abstract outputs. You can also adjust the other settings and parameters to complement the number of steps and improve the output.
These are some of the tips and recommendations for finding the optimal number of steps for your image generation goals. We hope they will help you master the art of stable diffusion steps and generate amazing images from text. In the next section, we will conclude the blog post and provide some links and resources for further learning or feedback.
Conclusion
In this blog post, we have explained what steps are and how they affect the image generation process in stable diffusion. We have also shown you some examples of images generated with different numbers of steps and compared their quality and diversity. We have also discussed some of the challenges and limitations of using steps in stable diffusion, and given you some tips and recommendations for finding the optimal number of steps for your image generation goals.
We hope you have learned something new and useful from this blog post, and that you are now more confident and curious about using steps in stable diffusion. Stable diffusion is a powerful and flexible technique that can help you generate amazing images from text prompts, but it also requires some experimentation and adjustment to get the best results. We encourage you to try stable diffusion and experiment with different numbers of steps and see what kind of outputs you can create.
If you want to learn more about Stable Diffusion and its settings and parameters, you can check out some of the following links and resources:
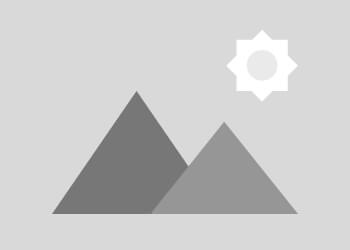
As intrepid explorers of cutting-edge technology, we find ourselves perpetually scaling new peaks. Today, our focus is the user interface of the open-source project, Automatic1111. If you’ve dabbled in Stable Diffusion models and have your fingers on the pulse of AI art creation, chances are you’ve encountered Automatic1111. Its power, myriad options, and tantalizing dropdown menus…
If you have any questions, feedback, or suggestions about stable diffusion and steps, you can leave a comment below. We would love to hear from you and see your creations. Thank you for reading this blog post and happy image generation!
Understanding Stable Diffusion
- What is Stable Diffusion?
- Is Stable Diffusion real?
- What do steps do in Stable Diffusion?
- What is the latest Stable Diffusion?
- How many people use Stable Diffusion?
- Is Stable Diffusion easy to use?
- What is similar to Stable Diffusion?
- What is Stable Diffusion style?
- How does Stable Diffusion training work?
- Is Stable Diffusion Pretrained?
- Can Stable Diffusion generate 3D models?
- How do you use Stable Diffusion at home?
- What is the new version of Stable Diffusion?
- What are the applications of Stable Diffusion?
Leave a Reply